Abstract
Over the past few years, there has been a growing interest in this field of Artificial Intelligence (AI). This review paper aims to examine the extent to which AI has been adopted across various domains in different regions of the world. Specifically, this study seeks to explore the effectiveness of AI in areas such as banking, governance, judiciary, and agriculture. While AI’s impact on these areas has been studied before, this research focuses on aspects that have not been given much needed attention so far. The study will begin by offering an overview of AI’s development from a regional perspective, analyzing 63 articles from leading journals and institutions to identify the most commonly used terminologies, theories, and technologies. The increasing computational power has played a crucial role in the advancement of AI. However, the role of AI in governance, judiciary, and legislature system has not been explored in depth in previous research. Furthermore, most studies tend to focus on single domain, whereas this paper aims to examine several domains and their relationship to AI. The study presents several AI-driven techniques, including Deep Neural Net (DNN), Artificial Neural Net (ANN), Candlestick Plots, Natural Language Processing (NLP), Explainable Deep Neural Net (xDNN), Attentional Maps, Digital Inventory, Deep Learning among others, that can be applied to enhance society’s performance in different domains. The research also delves deeper into the judiciary domain, proposing specific AI techniques that could be useful in this field. Additionally, tables summarizing different AI tactics relevant to each domain are presented.
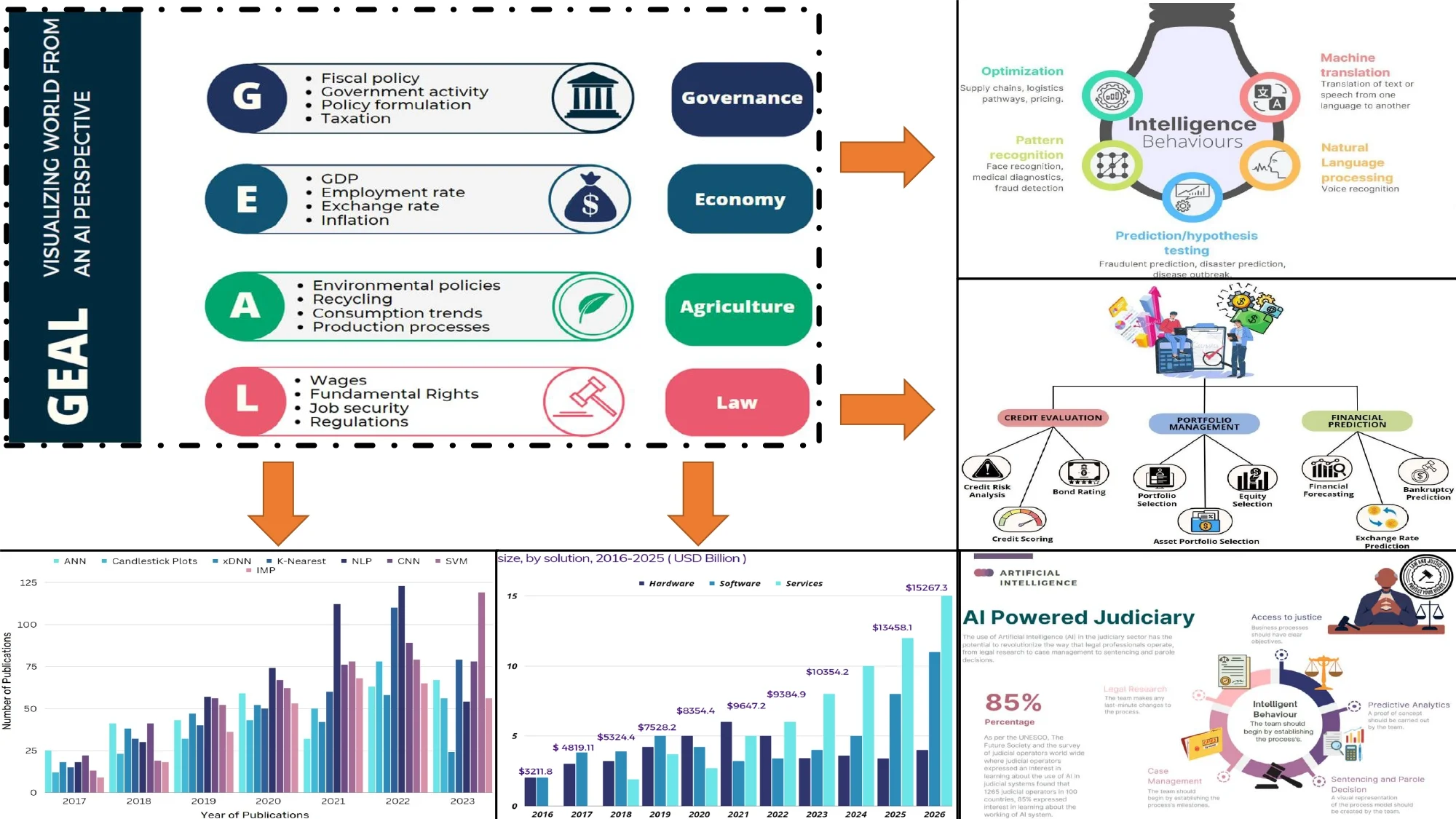
Highlights
- Data Privacy and Security: AI enables financial institutions to process vast amounts of data quickly and accurately. AI-powered chatbots and virtual assistants enhance customer experiences in the finance sector.
- AI systems can efficiently sift through large volumes of legal documents, case laws, and statutes to assist lawyers and judges in their research. Natural language processing (NLP) allows these systems to extract relevant information and provide insights that aid in preparing cases and making well-informed decisions.
- Fraud Detection: AI algorithms can analyze large datasets to identify patterns of fraudulent activities in government programs, tax evasion, and other illicit behaviors.
- By analyzing historical case data, AI algorithms can predict the potential outcomes of legal cases, which can be valuable for attorneys, judges, and litigants.
1. Introduction
The 20th century witnessed a significant shift towards the adoption of advanced technology in all sectors, replacing the traditional manual labour practices of the past. It was during this period that the term “artificial intelligence” was coined by John McCarthy in 1956. This happened in response to a query posed by a student at Dartmouth College who asked if there existed a machine that could learn to perform mathematical tasks. It is prominently fascinating to turn the pages of history and go back in the past glance and see how many ideas that were dreamt about in the past are coming true today. Incredibly, technology has advanced to a point in human history where practically every aspect of society, including the legal system, the agricultural sector, and even education, is undergoing a radical upheaval. Artificial Intelligence is widely discussed topic, with different opinions on its possible effects on society as we know it today [1]. As the use of artificial intelligence (AI) continues to expand, so does our ability to see the world through its lens. With the help of machine learning algorithms, AI can now process and analyse vast amounts of data, transforming our understanding of everything from traffic patterns to climate change [2]. But the true power of AI lies in its ability to visualize this data in new and compelling ways, allowing us to see patterns and insights that were previously hidden. Interestingly, AI's development has led to a radical upheaval in practically every aspect of society, including the legal system, agriculture, and education. While the effects of AI on society are hotly debated [3], AI has proven effective in domains such as finance, healthcare, e-commerce, transportation, governance, judiciary, legislature, and banking. Surprisingly, AI's impact on humankind is predicted to be more revolutionary than that of fire and electricity The various domains in which AI is revolutionizing include Finance, Healthcare, Education, E-commerce [8], Agriculture [4], Transportation [7], Banking, Governance Policy Formulation, and many more. The study of AI's effectiveness in governance and judiciary, which have not been extensively explored, is gaining attention.
Fig. 1AI-driven domains are covered in this review paper
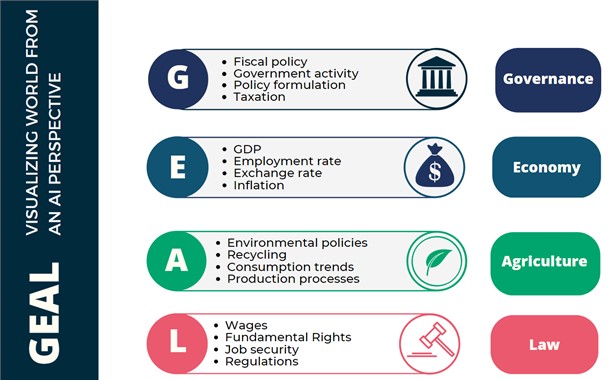
Artificial Intelligence (AI) has become an increasingly important topic in recent years, as it has the potential to revolutionize various sectors of human society. With its practical intervention, AI can help us achieve greater efficiency, accuracy, and productivity in areas such as governance, banking, agriculture, judiciary, and healthcare [10]. The application of AI in these sectors has the potential to transform the way we live, work and interact with each other. One of the most promising areas where AI can make a significant impact is in governance. The integration of AI in governance can improve service delivery, increase efficiency, and enhance citizen participation. AI can help governments identify areas of inefficiency and optimize their processes. For example, AI can be used to analyze data from traffic cameras to optimize traffic flow, or to analyze data on energy consumption to reduce waste and improve sustainability [36]. The banking sector has been one of the earliest adopters of AI technology, with applications such as fraud detection and personalized marketing. However, recent developments have shown that AI technologies are being integrated into a range of sectors, including transportation, health care, education, agriculture, and manufacturing. In agriculture, AI has the potential to revolutionize the way farmers work by helping them make better decisions and improve crop yield [52]. AI can help farmers analyze data on soil quality, weather patterns, and crop yield to optimize their farming practices. This can lead to higher crop yields, lower costs, and increased sustainability [23]. The judiciary is another sector where AI can make a significant impact. AI can help expedite legal proceedings, reduce errors, and enhance access to justice. For example, AI can be used to analyze legal documents and identify relevant case law, which can help judges make more informed decisions [34]. AI can also be used to predict the outcomes of legal cases, which can help lawyers make better decisions on behalf of their clients.
The study considers 66 articles in terms of leading journals, institutions, and the most frequently used terminologies, theories, and technologies adopted. Since strong AI is still a relatively new idea, its lack of adoption across different industries is a cause for concern. While existing literature has focused more on the banking sector, the primary goal of this review paper is to highlight several AI techniques that can be used in a variety of areas of human society. The survey also attempts to introduce numerous modus operandi strategies that are essential in every area of a developing society, as well as to guide the reader through the entire artificial intelligence vocabulary with simplicity [37]. In conclusion, AI has the potential to transform several sectors of the economy including governance, economy, agriculture, and judiciary. By leveraging the power of AI, we can enhance productivity, optimize processes, and improve decision-making, ultimately leading to a better quality of life for people around the world [51]. The study of AI is a rapidly evolving field, and as such, it is important to remain up-to-date with the latest developments and technologies to ensure that we can fully leverage the potential of AI for the betterment of humanity.
Fig. 2AI-powered toolbox
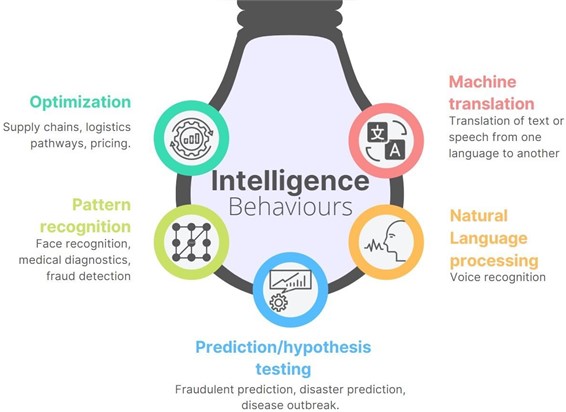
Artificial intelligence (AI) is a force for good as it has the potential to revolutionize various sectors of human society. AI can help humans reach their full potential by augmenting human capabilities and improving decision-making processes [26]. For instance, the use of AI in governance can improve service delivery, increase efficiency, and enhance citizen participation [37]. Similarly, the use of AI in the economy can help businesses make data-driven decisions and improve productivity. AI can also play a significant role in the agricultural sector by helping farmers make better decisions and improving crop yield [29]. In the judiciary, AI can help expedite legal proceedings, reduce errors, and enhance access to justice. However, the development and implementation of AI also raises ethical concerns (UNESCO 2021). The impact of AI on society and the environment needs to be evaluated and managed to ensure that the benefits of AI are realized while minimizing the risks [43]. In conclusion, AI has the potential to transform several sectors of the economy including governance, economy, agriculture and judiciary. By leveraging the power of AI, we can enhance productivity, optimize processes, and improve decision-making, ultimately leading to a better quality of life for people around the world.
2. Meta analysis
A dearth of literature on artificial intelligence (AI) covers many areas of the public sector. For this reason, specific contextualized publications were chosen and examined by searching on Google Scholar for the keywords “Artificial Intelligence”, “AI impact on diverse domains,” “Evolution of AI”, “The increasing importance of AI,” and “Artificial Intelligence in Public Administration.” A total of 239 papers with publication dates between 2010 and 2023 were found throughout the search. The value or relevance of each document to the literature was examined first, followed by the titles and abstracts of each document, and then the content, which included reading the document and filtering and organizing keywords. The advancement of machine learning techniques has resulted in a surge of publications and the emergence of new sub-fields, leading to expansion of AI. The volume of articles published every year demonstrates this growth, as depicted in Fig. 3. The occurrence of conference every two years affects the number of publications, impacting the outcomes. The number of AI publications has been steadily increasing in the 21st century, with the growth rate of journal articles distant from that of conference articles.
Average author self-reference rate declining from over 40 % at the start of twenty century to only 10-15 % at the end of the decade. Some researchers recognized the need to explain AI/ML model-based intelligent systems and began investigating and proposing approaches. The term explanation became associated with expert systems in the mid-2000s, eventually evolving into a vast research field known as explicable artificial intelligence (XAI). However, until 2017-2018, XAI received little attention from academics, as evidenced by the yearly trend of publications containing the phrase “explainable artificial intelligence” in their titles or abstracts across several bibliographic databases.
Fig. 3a) Outlines the trend in publication volume from 2010 to 2023; b) From 2018 through March 2023, a specific number of articles are highlighted
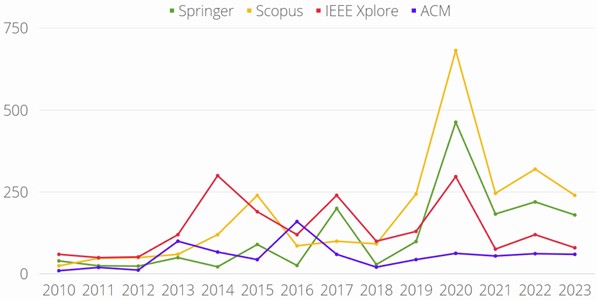
a)
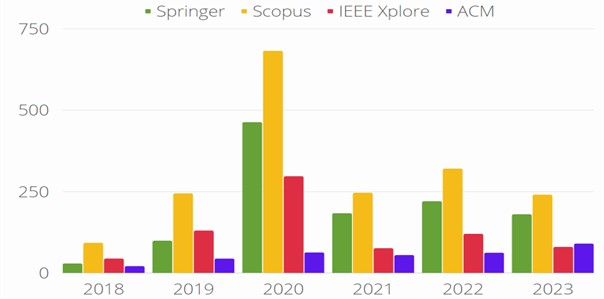
b)
Several researchers have acknowledged the importance of explaining intelligent systems based on Artificial Intelligence/Machine Learning (AI/ML) models, and they have been working on various approaches for this purpose for quite some time. The idea of explaining AI/ML models gained more attention in the mid-2000s when a connection between expert systems and the term explanation was documented in bibliographic databases. Despite this, until recently, XAI had received little attention from academics, as evidenced by the annual trend of publications from several bibliographic databases that contained the phrase “explainable artificial intelligence” in their titles or abstracts. However, the field of XAI has gained more traction in recent years, particularly in 2017-2018, as researchers recognize the importance of transparency, accountability, and interpretability in AI/ML models. Several techniques, such as decision trees, rule-based systems, and model-agnostic approaches, have been proposed to make AI/ML models more transparent and interpretable. XAI has the potential to enhance the trustworthiness, usability, and ethical standards of AI systems, making them more accessible to diverse groups of stakeholders, including policymakers, end-users, and the general public.
Fig. 4Percentage of publications on various XAI techniques for various applications: a) domains and b) activities
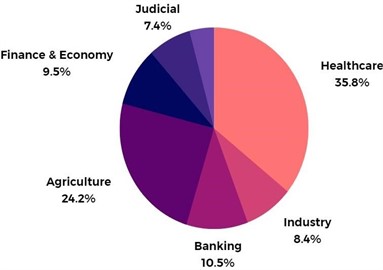
a)
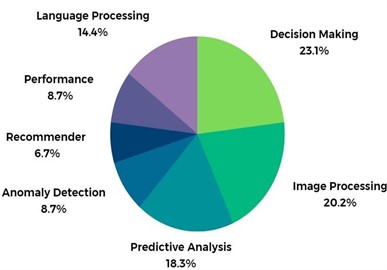
b)
Academics who use AI/ML models have recently shown increased in XAI, which can be attributed 3 significant events. The DARPA-funded “XAI Program” was launched at the beginning of 2017, followed by the Chinese government’s publication of the “Development Plan for a New Generation of AI” in mid-2017, and these of the “General Data Protection Regulation (GDPR)” in mid-2018, which granted the European Union people a “Right to Explanation” for algorithmic decision making. The impact of these events is reflected in the increasing number of XAI-related articles published in significant bibliographic databases. As can be shown in Fig. 4(b), XAI is highly required for decision support tasks in various application fields where AI and ML technologies are applied for decision support systems.
3. Market size
AI is already being used in industries such as finance, national security, healthcare, criminal justice, transportation, and smart cities, and is expected to continue growing at a CAGR of 20.1 % from USD 387.45 billion in 2022 to USD 1394.40 billion in 2029 [40]. The increasing demand for AI technology across numerous industry verticals, including retail, BFSI, healthcare, food and beverage, automotive, and logistics in driving the global market expansion. AI provides enormous prospects for economic growth and is estimated to perform 80 % of all American professionals’ employment by 2020 [46]. AI and algorithmic data together could enhance India’s economic growth, and by 2025, data and AI might boost India’s GDP by 450$ to $500 billion [41]. According to PriceWaterHouseCooper research, AI technology may enhance global GDP by $15.7 trillion, or 14 % by 2023. China has invested $150 billion in AI and is advancing rapidly to become a worldwide leader [45]. The market size of artificial intelligence (AI) varies across different domains in a developing nation. The use of AI in healthcare has been growing rapidly in recent years, and the market size of AI in healthcare is expected to reach $34 billion by 2025 globally. AI in governance has the potential to transform governance by improving efficiency and transparency in government processes. The market size of AI in governance is expected to grow from $8 billion in 2020 to $32 billion by 2025 globally. AI can help improve the efficiency and accuracy of legal processes, and the market size of AI in judiciary is expected to grow from $317 million in 2020 to $1.1 billion by 2025 globally. AI has the potential to transform the economic sector by improving productivity, reducing costs, and enabling new business models. The market size of AI in the economic sector is expected to reach $13 trillion by 2030 globally. AI can help optimize crop yields, improve soil health, and reduce the use of harmful chemicals in agriculture. The market size of AI in agriculture is expected to grow from $500 million in 2020 to $4 billion by 2026 globally. Overall, the market size of AI in developing nations varies across different domains, but is expected to grow significantly in the coming years as organizations adopt AI-powered tools and systems for various applications.
Fig. 5Artificial intelligence market size, share & trends analysis report by solution, by technology (deep learning, machine learning, natural language processing, machine vision), by region, and by segment forecast, 2016-2025
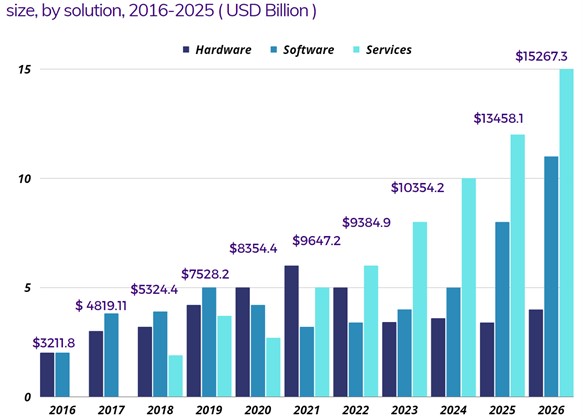
4. Algorithmic world
AI, a branch of computer science, deals with intelligent machines and has its roots in logic, mathematics, and philosophy. Its foundations were laid in the mid-20th century with the invention of neural networks by John Hopfield and Marvin Minsky [40]. IBM's Deep Blue Chess computer led to significant growth in AI research during the 1980s. AI encompasses anything from computer programs to self-awareness and can perform a range of intelligent behaviours such as optimization, hypothesis testing, pattern recognition, facial landmark detection, and natural language processing [62]. AI fuelled by higher computational power can lead to better connectivity and economic growth. Its applications can accelerate scientific progress, medical diagnosis, agricultural productivity, supply network streamlining, and governmental decision-making [35].
4.1. Governance
Governments around the world are embracing AI to solve various issues with public policy frameworks. The US, UK, China, Mexico, and the European Union have all put AI approaches into practice to enhance citizen relations and create various government policies. The machine learning algorithm's ability to forecast outcomes properly will increase with the amount of data input into the system of government and public administrative databases, and decision making will become more sophisticated [63]. Good governance involves people coming together to reach sound decisions for the benefit of the public. It is crucial for both socioeconomic progress and an efficient system of governance. Probity is an essential element of good governance, and it entails acting with honesty, decency, and integrity [64]. Mexico has developed a national AI policy and allied with some of the most technologically proficient nations globally, becoming the first country in Latin America to declare a national AI strategy [65]. The US has established the National Artificial Intelligence Advisory Committee (NAIAC) to administer and ensure the integrity of National AI governance. AI can play a significant role in various aspects of government policy formulation and administration. One of the primary ways in which AI can be utilized is through the analysis of vast amounts of data to identify patterns and trends that can inform policymaking. In terms of administration for example, AI-powered chatbots can provide citizens with instant assistance, reducing the need for manual intervention. Additionally, AI can be used to detect fraud and other forms of financial crime improving the integrity of government programs and ensuring that funds are used appropriately. AI can also be utilized to improve financial inclusion, which is the process of providing access to financial services and products to individuals and communities that are currently unreserved, AI-powered tools can be used to assess creditworthiness, identify potential risks, and tailor financial products to specific needs. This can help individuals and communities access credit, save money, and build wealth which can lead to long-term economic growth and stability.
Fig. 6Governance is the exercise of economic, political, and administrative authority to manage a country’s affairs on all fronts
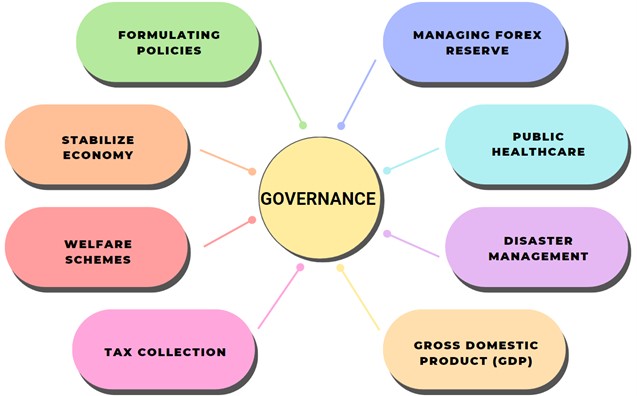
Artificial intelligence (AI) can manage massive datasets and make quick decisions, which is how it can deal with starting IPOs and stabilising the rate. The number of IPOs will raise the amount of share buying and selling, which will grow corporate capital. More resources equate to more large-scale production and employment. People will put more of their money into IPOs and less of it into gold and inflation index bonds IIBs, which will lead to a decrease in the import of gold and a reduction in the current account deficit. The Gross Domestic Product GDP of a country can therefore be benefitted. The following illustration (Fig. 7) depicts how AI-powered techniques, including K-means clustering, dimension reduction, machine translation, natural language processing, convolutional neural networks, artificial neural networks, and pattern recognition, can enhance policy formulation, decision-making, and various other government tasks [59]. Consequently, there is less room for errors in AI-driven methodologies [60]. Humans, on the other hand have a tendency to forget factors and lose focus, limiting their ability to make sound decisions [54]. The fundamental differences between AI and human decision-making are the accuracy with which AI-powered algorithms consider multiple parameters and process data to generate results [58]. Our limitations as humans to remember everything and maintain focus make us inferior to AI in decision-making.
Fig. 7Various techniques can be used in various provinces of AI-powered Governance
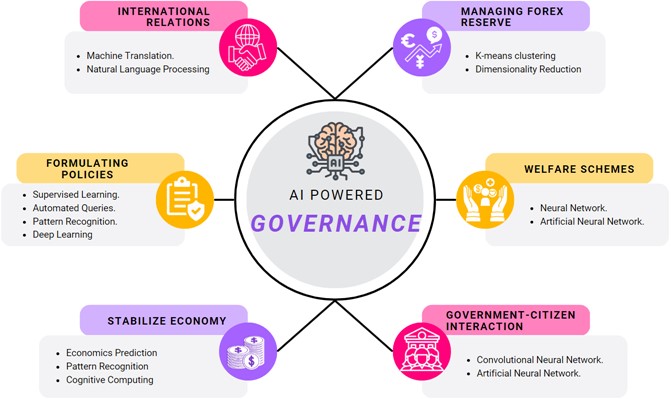
The government has developed AI for a variety of purposes, including transportation and reducing criminal risk. For instance, [53] uses artificial neural networks, a type of AI, to explore crime risk prediction as a factor that leads to safer travel in metropolitan settings. Using the database of the Chicago Police Department, the writers compiled crimes that occurred in the city of Chicago. After this data was clustered, “artificial intelligence was utilized to develop and compare neural network forecasting models to determine the best neural network model” (page 469). To prevent crime or to develop public measures to counter these dangers, the outcome of this research was the ability to anticipate some high-crime-risk transportation regions. After testing several neural network models, the author concludes that AI is useful and effective in the battle against crime.
4.2. Economy
For the financial industry, artificial intelligence is equivalent to the invention of fire for a caveman. In general, uncertainty is the only known certainty when it comes to predicting or forecasting specific parameters in the financial arena. Thus, it follows that economists tend to make poor predictions. Since AI can comprehend enormous amounts of data and sort important facts in a finite amount of time, this capability is unimaginable to humans. Every economist in the world had considered AI to be a game-changer for the discipline of economics. In the world of banking and financial services, major corporations view artificial intelligence as an indispensable technology [36]. The economic sector is, therefore, the superior sector. Despite being the most effective at making predictions and interpreting data, artificial intelligence struggles with the tension between opacity and transparency. Given that AI is a “black box,” understanding its internal workings is impossible, which raises the question of whether we should entrust AI with making difficult decisions because it won't be able to defend its actions [1].
Making credit and other financial products available, forecasting financial trends, simulating investor behavior, goal evaluation, asset portfolio management, pricing initial public offerings, determining the optimal capital structure, spotting patterns in security price movements, and forecasting defaults and bankruptcy are just a few of the ways that researchers have attempted to assist the financial sector [4] and [25]. Before the advent of artificial intelligence, individuals employed soft computing techniques, decision trees, discriminant analysis, logistic regression, and other parametric and nonparametric statistical techniques (e.g., artificially intelligent algorithms and rough sets). Currently, the financial markets sector uses artificial intelligence approaches (particularly ANN) the most and has made them the most popular instrument. While Neural Networks (NN) have attracted greater attention over time due to their independence from data distribution assumptions, capacity for self-updating, and primary status as an open-source tool. As a result, NN is preferred over these nominal statistical techniques when using plain data as the input. Four domains can be taken into consideration while applying artificial intelligence to the financial markets. Here are those domains: (A) credit assessment, (B) portfolio management, (C) financial forecasting, and (D) security of financial assets.
As human selection is expensive, AI and ML are replacing human analysts in corporate tasks. Since AI is built on ML, it learns over time and ensures the utmost accuracy in calculations and data analysis. AI-powered Chat-Bots have established themselves as viable customer service tool, and because they save businesses time and money, they appear to be an uncompromisable resource. Non-commercial activities would likewise change as a result of this ground-breaking technology (Maruti tech labs, 2019) [24]. Banks and other financial institutions must be quick while collecting and disbursing interest on deposits and dividends since they deal with deposits and payments. This is why banking and financial organizations view fraud as a severe problem and use AI-enabled technology to prevent financial pollution [1]. If it raises a red signal for regular transactions and that flag is later cleared by a human, AI enables the system to learn from that experience and make wise judgments about what should be classified as fraud and what shouldn’t [16].
Fig. 8Several attributes of Financial Markets can be augmented with the help of Artificial Intelligence tools and techniques
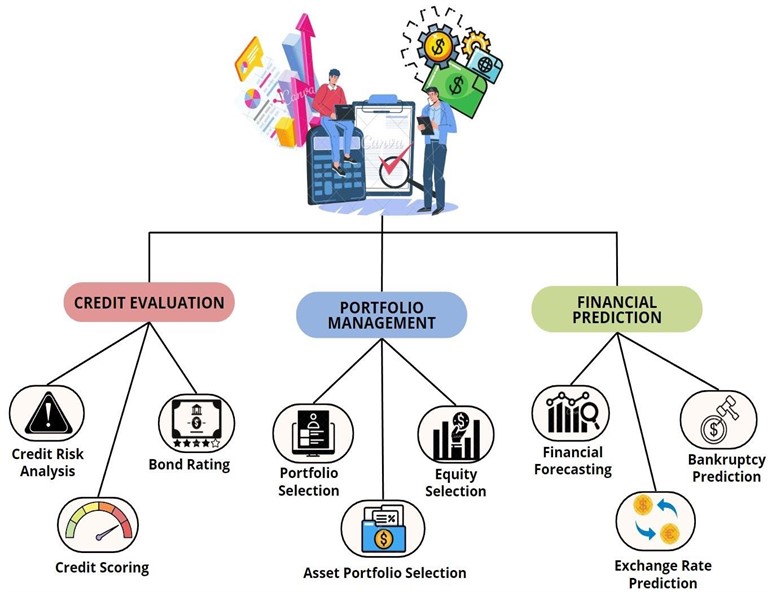
The financial sector faces several challenges, including those related to credit scoring, bond rating, credit risk analysis, portfolio selection, asset portfolio selection, equity selection, financial forecasting, exchange rate prediction, and bankruptcy prediction [28]. In general, 5 Neural Network models can be used to address these challenges. Multi-Layer Perceptron (MLP), Radial basis function, Learning Vector Quantization, and Fuzzy Adaptive Resonance are the five advanced and high-performance computing neural network models in charge of making up for these losses [32]. Linear Discriminant Analysis (LDA), Logistic Regression, K-Nearest Neighbour, Kernel Density Estimation [36], and Decision Tree Model [39] were among the numerous outdated conventional techniques that were formerly utilized explicitly.
Fig. 9Numerous techniques have the potential to escalate the economic sector’s position to a new height
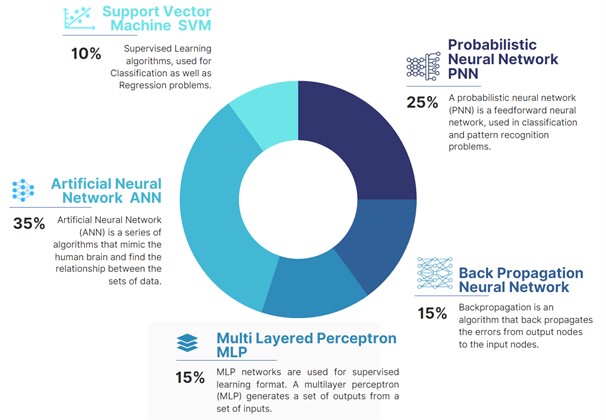
Support Vector Machines (SVM), Artificial Neural Networks (ANN), Multi-Layer Perceptron (MLP), Backpropagation, and Probabilistic Neural Networks (PNN) are all machine learning techniques that have the potential to revolutionize the economic sector by providing accurate predictions and insights. SVM is a powerful classification algorithm that can be used to analyze large datasets and identify patterns that are not immediately visible to the naked eye [31]. It has been used to identify and predict market trends, stock prices, and consumer behaviour. ANN, MLP, and Backpropagation are all neural network-based techniques that have been used extensively in the financial sector [32]. They have the ability to identify patterns and relationships in large datasets and provide predictions for various economic indicators such as inflation, GDP growth, and interest rates. Additionally, these techniques have been used in the identification of credit card fraud and money laundering activities, thus aiding in financial risk management [38]. Probabilistic Neural Networks (PNN) is a statistical classification algorithm that can be used to identify patterns in economic data [37]. It is particularly useful in applications where the data is uncertain, such as in predicting bankruptcy or default risk in lending, or in assessing the likelihood of a successful investment opportunity.
Table 1This table lists several financial market issues as well as some AI-inspired preventative strategies
Problems | Suitable AI-technique |
Credit scoring | Probabilistic neural network |
Credit risk classification | Decision tree |
Corporate credit rating | Support vector machine |
Bankruptcy prediction | Artificial neural network |
Stock Market return forecasting | Artificial Neural Network |
Exchange rate forecasting | General Regression Neural Network |
Bank Financial Failure prediction | Multi-Layered Perceptron |
Equity profitability | Back-propagation neural network |
In summary, the use of SVM, ANN, MLP, Backpropagation, and Probabilistic NN in the economic sector has the potential to provide valuable insights and predictions that can help businesses, policymakers, and investors make more informed decisions [42]. As data continues to grow in volume and complexity, these techniques will become increasingly important in identifying and analyzing patterns in economic data.
[25] developed a support vector machine SVM that, when compared to ANN, primarily blocks and addresses the challenge in the field of credit rating analysis. The outcomes suggested that SVM and ANN function similarly. MLP-Neural Networks were employed by [55] as a prediction model, and they examined several well-known feature classification techniques.
4.2.1. Know your customer
KYC is an important operation that essentially provides the bank with all the essential data needed as input. KYC informs the bank of its lenders and investors. There is a potential that the investor could participate directly or indirectly in illegal activities including money laundering, financing terrorism, using black money, and other financial crimes if the KYC process is not enforced. Therefore, these KYC records are provided as Customer Due Diligence CDD, and billions of records are handled and tracked. For an average human being, maintaining and verifying records regularly is so difficult. This makes some new technical modifications to the system necessary right now. When there are many transactions with varying patterns, AI is an excellent technique for detecting aberrant patterns and identifying regions of danger. Machine learning can be used to analyse transactions and can spot many types of suspicious investment behaviour. The voice data of the con artist can be read and sent to artificial intelligence for processing with the aid of natural language processing. From the output of NLP, artificial intelligence will extract the metadata. As a result, the extracted metadata can be examined further, and further information about the con artist will be noted. Figure 11 depicted above is a pie chart that describes various AI techniques that can be harnessed to counterattack the problems of the Financial Sector.
4.2.2. Money laundering
A major issue facing the financial services industry is money laundering. The United Nations Office on Drugs and Crime estimates that the financial sector “cleans” an annual $2 trillion. Fine collections for banks that failed to keep an eye out for fraudulent investments have climbed “500 X” during the past ten years. Because of this, banks have established a syndicate to handle the time-consuming work of keeping a careful check on questionable transactions, which frequently causes numerous tiny branches to suffer concussions. Finding questionable transactions and networks of transactions is possible with the aid of machine learning algorithms used in anti-money laundering. These transactions are marked for inquiry and given a high, medium, or low priority rating so that the investigators can divide their time between the most important transactions and the least important ones. To avoid trends that don't result in money laundering, AI can also learn from the investigators as they analyse and approve questionable transactions and automatically reinforce the model's understanding.
4.2.3. Fraud detection
The banking industry has serious difficulty with fraud detection. Given the wide range of fraud forms and the number of transactions that need to be evaluated, which manual human-based and rule-based systems can’t keep up with, detecting and preventing fraud is a hugely structured task for banks. AI can analyse massive amounts of data to look for fraud patterns, and then utilize those patterns to spot fraud as it occurs in real-time. AI models can be used to reject transactions outright when fraud is suspected or to flag such transactions for further examination. To help investigators prioritize their work, an AI-based system will generate a score for each scam and grade it according to its level of threat.
Fig. 10Several attributes of financial markets can be augmented with the help of artificial intelligence tools and techniques
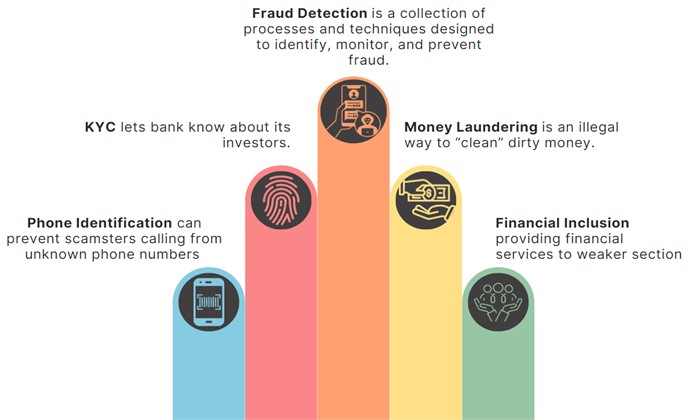
4.2.4. Financial inclusion
Financial inclusion refers to deepening the roots of financial services. It is frequently noted that people in rural areas frequently become entangled in the web of greed. Because of this, they disregard the terms and conditions and accept the payment without hesitation. Such individuals are specifically targeted and even provided several inappropriate schemes. When the money lenders catch these folks, they raise their interest rates and add additional fees. As a result, these individuals adopt various actions including turning into thieves, starting businesses based on looting, engaging in smuggling, and even engaging in prostitution, all of which have a negative influence on not only their own lives but also the lives of their families. They merely perform such actions to pay back their loans. However, as time goes on, they are subjected to increased interest rates and eventually fall into an endless cycle of debt, leaving them with no choice but to commit themselves. This is the main cause of the terrible necessity for AI use in this province. Financial services powered by AI could be widely available in rural provinces, effectively putting an end to the unsuitable money lending industry.
4.3. Agriculture
Artificial intelligence is one of the key areas of research in this cutting-edge technical field of computer science. Due to its rapid technological breakthroughs and broad application to issues that are typically difficult for both standard computing systems and humans to effectively solve (E.Rich and Kevin Knight., 1991), this technology is spreading swiftly. Similar to other fields of high significance, farming occupies around 30.7 % of the world's population or 2781 million hectares of land [16]. As a result, from planting to harvest, farmers must overcome many obstacles. Agriculture is regarded as the foundation of a country’s economy. For developing countries, agriculture serves as a backbone for their socioeconomic development. Only the agricultural sector is to be blamed for long-term financial expansion and economic escalation. By 2050, there will be over 9 billion people on the earth, according to the Food and Agriculture Organization of the United Nations. A new paradigm in agricultural production is being compelled by issues like rising population, shrinking farmland, decreasing natural resources, unexpected climate change, and changing consumer demands. The new agricultural system needs to be more productive in terms of output, operational efficiency, resistance to climate change, and sustainability for future generations. The capabilities of AI in dealing with the problems presented by this new paradigm are astounding.
Fig. 11Depicts several AI models that can be utilized to tackle the problems of the Agricultural domain. The most widely used AI computational model is ANN
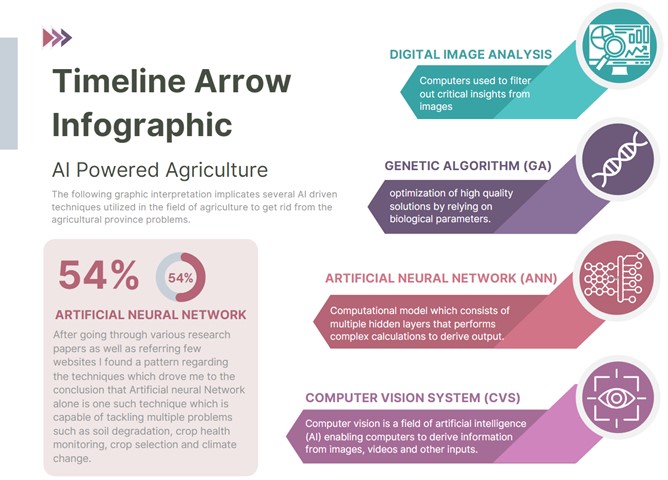
There are many options and unknowns in farming. Farmers must manage these unknowns. Since agriculture is an evergreen industry, it frequently has issues. This paper will only touch on the issues since if every issue were taken into account, it might be next to impossible to determine the paper's goals and objectives. Soil degradation, crop selection that takes into account environmental circumstances and other aspects, weed monitoring, pest control, and climate change are issues that are encountered in the agricultural sector. As a result, AI-driven tools and procedures can protect against the majority of the agricultural province’s fundamental issues. Support Vector Machine, Digital Image Analysis (DIA), Learning Vector Quantization (LVQ), Artificial Neural Network (ANN), Optimization utilizing Invasive Weed Optimization (IWO), Fuzzy Xpest, Computer Vision System (CVS), Genetic Algorithm (GA), Fuzzy Logic SRC, and Fuzzy Cognitive Mapping are some examples of AI-based techniques.
4.3.1. Soil management
A crucial component of agricultural activity is soil management. A farmer who is well-versed in the many varieties of soil that are accessible would undoubtedly do better than other farmers. Similar to how good knowledge of different soil types can improve crop productivity, passing along proper insight can also protect the soil's inert nutrients, resulting in soil conservation. A conventional soil survey approach can be used to assess if urban soils contain contaminants [16]. The knowledge acquisition, conceptual design, and system implementation phases make up the Soil Risk Characterization Decision Support System (SRCDSS) construction process [19].
4.3.2. Crop management
Crop management starts with seeding and progresses through monitoring growth, harvesting, crop storage, and distribution. It can be summed up as actions that increase the yield and growth of agricultural products. Precision crop management (PCM) is essentially an agriculture-based management methodology that places a strong emphasis on improving crop productivity and profitability. Precision crop management focuses on boosting productivity and protecting the environment by taking into account many elements that have a direct impact on the soil. The lack of timely, widely disseminated information on crop and soil conditions has impeded PCM [21]. The only way to address issues with crop health, production, and water management is to combine several agricultural management systems. A flexible strategy should be taken into account during this procedure. When evaluating cropping options, consideration should be given to the timing, intensity, and predictability of drought [25]. When the weather pattern is understood, deciding what crop to plant to maximize agricultural production will be greatly influenced. If seen from a farmer's perspective, a little awareness of weather patterns can greatly decrease their concussion and aid with decision-making [22]. A combination of hardware and software is used when it comes to artificial intelligence. The list of elements used in this method includes the following: autonomous vehicles, Sensors, rotary and linear actuators, a manipulator, an end-effector, a computer vision system, and a three-dimensional Visualize the fruit and the setting. Finally, a control strategy that creates collision-free motions for the manipulator during harvesting [29]. For each site, rainfall information and weather variables can be used. Predictions of rice yield are more accurate when ANN parameters are changed. Less hidden nodes and slower learning rates in model optimization were needed for smaller data sets [34].
Fig. 12Represents a pie chart that indicates various sub-domains that can be nourished with the help of Artificial Intelligence powered technique
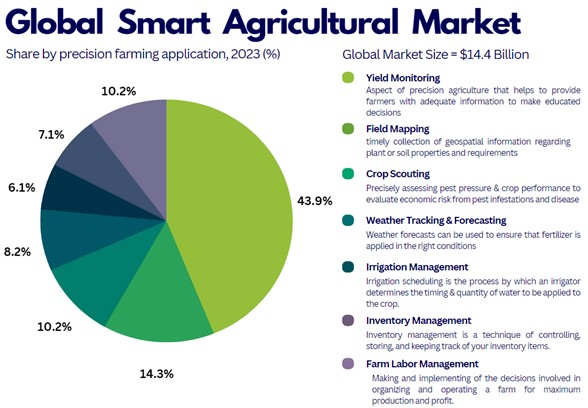
4.3.3. Disease management
In agricultural regions, disease control is extremely important. Plant disease is one such important element that adversely affects agricultural yields. These diseases, which affect both plants and animals, are caused by a variety of factors, including genetics, soil type, rain, dry weather, wind, temperature, and others. Due to these numerous variables and the nonlinear character of a select few illnesses, this feature presents a tremendous difficulty, particularly in the context of industrial-scale farming. A farmer should adopt an integrated disease control and management approach that incorporates physical, chemical, and biologically inspired preventive strategies to effectively control such diseases and minimize losses [9]. Such an integrated system takes a long time to develop and is not at all cost-effective (Weed Science Society of America, 2020). The capacity of a text-to-talking user interface is provided by a text-to-speech (TTS) converter. It offers a highly efficient user interface for live interactions on the web [34].
4.3.4. Weed management
Farmers frequently face issues with predicted profit and production, and one of the main causes is the lack of early weed treatment. If weeds are not treated early, they can cause dryness and destruction of maize crops, resulting in a 50 % from in yield. Weeds also reduce wheat output by about 48 %, and sometimes even up to 60 %, due to weed competition. Soyabean yield can be reduced by 8 % to 55 % because of weed effects. While weeds can have some beneficial effects, such as clearing the way during wildfires, they often outcompete plants or crops for essential resources like water, nutrients, and sunlight, causing significant harm. The use of Unarmed Aerial Vehicles (UAVs) can be an effective way to address these issues. UAVs can capture images of crops, which are then converted into binary images, segmented, and processed to detect crop rows and classify weeds. AI has made full potential in this field. More still needs to be done to improve agricultural activities using AI as there are many limitations to its implementation.
4.4. Judiciary
The use of artificial intelligence (AI) in the judiciary has the potential to revolutionize the legal system, providing faster and more accurate decisions while reducing the cost of legal proceedings. AI has the ability to process vast amounts of data quickly and objectively, making it an attractive option for analyzing complex legal cases. However, there are also concerns regarding the use of AI in the judiciary, such as the potential for bias and the lack of transparency in decision-making. This review paper will explore the advantages and challenges of implementing AI in the judiciary, as well as the ethical considerations that need to be taken into account when using these technologies [34]. Through an analysis of current research and case studies, this review aims to provide a comprehensive understanding of the potential impact of AI in the legal system, and to offer insights into how these technologies can be used to promote fairness, efficiency, and access to justice [17]. AI systems can also learn from past decisions, which could help to reduce errors and inconsistencies in legal rulings. This could lead to more accurate and fair outcomes, especially in complex cases where human judges may struggle to process all of the relevant information [51]. AI can also help to ensure consistency in legal decisions by applying the same rules and criteria to each case. In addition, AI can increase access to justice by making legal services more affordable and accessible to people who might otherwise be unable to afford them. For example, chatbots or virtual assistants could be used to provide legal advice or guidance to individuals who cannot afford a lawyer. This could help to reduce the justice gap, where many people are unable to access legal services due to cost or other barriers.
Artificial intelligence (AI) has made enormous strides in recent years thanks to the creation of machine learning, deep neural networks, natural language processing, computer vision, and robotics. Lawyers predict that systems like Natural Language Processing, Deep Neural Networks, and Artificial Neural Networks, which can think like humans and make decisions, will soon replace current approaches and procedures used by courts and the judicial system [10]. Huge amounts of data are readily available, which has fuelled the development of artificial intelligence technology. The area of jurisdiction for justice administration has increased thanks to AI algorithms; as a result, Law, and Order, the branch of the judiciary, will see increased convenience in doing business [61]. Incorporating artificial intelligence into the judicial system could improve the quality of the services offered by the machinery, thereby raising the bar for legal professionalism [52]. By prioritizing the cases based on the major crimes committed, AI may even increase the effectiveness of the judicial database for the benefit of the general public [61]. As a result, the general public will have a stronger sense of trust in the judicial system, which may even result in a drop in crime. An integrated system known as Online Dispute Resolution (ODR) has been developed as a result of the partnership of Artificial Intelligence with online services (such as the www) [57]. With accuracy and speed that are unmatched by humans, artificial intelligence has a significant potential to increase the legal sector’s predictability, efficiency, and correctness.
Fig. 13Funnel illustration, company petitions, which make up just 1 % of all cases, are pending for 2,179 days on average, or almost six years
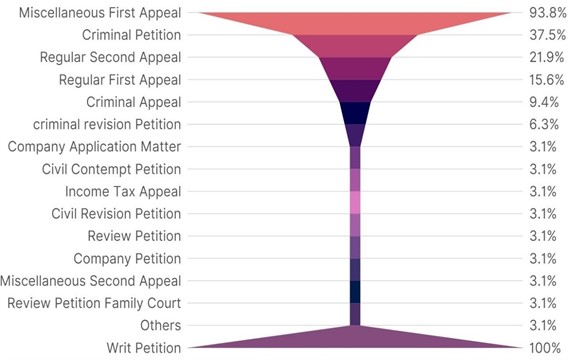
Combining AI systems and machine learning is not a futuristic idea; numerous existing technologies, including predictive justice and recidivism prediction in the criminal context, are already integrated into the functioning of the judiciary globally [52]. The judicial system is now being researched and is in its early stages of automation employing artificial intelligence and machine learning, although the court system has already been digitalized in many different ways around the world [10]. The European Court of Justice has already begun some level of digitalization, including e-filing, e-conferencing, and prediction algorithms (Paula C. Arias, 2020) [30]. Hangzhou, Beijing, and Guangzhou have already established a few internet courts, demonstrating China’s leadership in the judicial system's automation [10]. Artificial intelligence, blockchain technology, and big data are all used in the courts.
New software tools emerge bring with them both benefits and challenges. For instance, Ross was “employed” by an American law company in 2016 [17]. Ross was the first AI lawyer in history supported by Watson, IBM's cognitive computer. The AI tool (Natural Language Processing, NLP) enables human-language communication with lawyers, provides people with an opportunity to interact with potential employees, and can address specific bankruptcy consulting difficulties [44]. In the UK, a robot lawyer named Do-Not-Pay was developed to help consumers prepare legal documents and contest traffic citations. However, the development of artificial intelligence (AI) presents significant difficulties for the administration of justice and judicial systems [54]. Technology based on artificial intelligence (AI) will fundamentally alter how the legal sector operates. Most judicial tasks may be made simpler by AI, which has demonstrated potential in this area. The following are just a few of them: case push, knowledge index query, auxiliary conviction, sentencing reference, evidence review, and judgment, integrated support in court, voice/image reading and transformation, case management, deviation analysis, judicial/administrative connection, automatic document generation, and peer-to-peer proofreading.
Artificial Intelligence (AI) has the potential to revolutionize the judiciary sector in numerous ways. Firstly, AI-powered systems can significantly enhance legal research by scanning vast amounts of legal information and identifying relevant cases and precedents. This can save time and improve the accuracy of legal research. Moreover, AI-powered tools can also help judges and lawyers manage cases by tracking case-related information, managing deadlines, and automating routine tasks such as document management and scheduling. In addition to this, AI can also help predict the outcomes of legal cases based on historical data and trends, which can help lawyers and judges make more informed decisions. Predictive analytics can be used to develop tools that help judges make fair and unbiased sentencing and parole decisions by analyzing factors such as the offender's criminal history, risk of reoffending, and other relevant factors. Another key advantage of AI in the judiciary sector is the potential to increase access to justice. AI-powered tools can automate routine legal tasks, reduce the workload of lawyers and judges, and provide legal assistance to individuals who cannot afford a lawyer. This can help to ensure that justice is accessible to all individuals, regardless of their financial situation. Overall, AI can bring significant improvements to the judiciary sector, including enhancing legal research, managing cases more efficiently, predicting legal outcomes, ensuring fair sentencing and parole decisions, and increasing access to justice. With continued advancements in AI technology, we can expect to see further innovative applications of AI in the judiciary sector in the future.
Fig. 14AI-driven judiciary: the integration of artificial intelligence (AI) technology in the legal profession has the potential to improve efficiency, accuracy, and consistency in legal outcomes
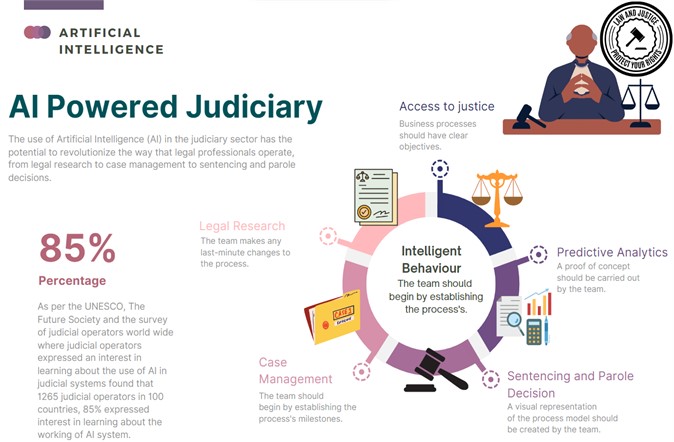
4.4.1. Challenges faced in judiciary
Information technology (IT) and artificial intelligence (AI) present both new potential and significant new obstacles for courts and judges. The management of judiciaries faces the biggest challenge. Judicial independence and case processing are the two fundamental determinants of governance, or how and by whom judgments are made. The goal of judiciaries is judicial independence. Both of these factors limit innovation potential. AI predictive models may have subtle data biases, which might be challenging to spot. Some options in AI prediction techniques may be the type of datasets to use, the data to include or exclude, the AI models or AI algorithms to choose, and the information to stress or underemphasize. These minor decisions taken together could result in different social groupings being treated more or less favourably. Due to this, the employment of such AI systems in decision making may quietly alter social and political power dynamics by automatically making decisions that are more favourable to some groups than others. AI has demonstrated its value in the legal system. However, there is no proof that judges will eventually be replaced by robots. Before AI can flourish in courts, there is still a lot of work to be done. Self-explanatory, a necessary element of reliable AI is currently not possible. Artificial intelligence can offer suggestions and counsel as legal knowledge becomes more complex. The main ethical concern is to determine how the use of AI may be changing fundamental legal principles and to make sure that these important principles are upheld during the technological transformation.
5. Result and discussion
A total of 67 technical papers, consisting of research papers, journals, conference papers and reference books, were reviewed in this study to analyse the penetration of AI in different provinces.
Table 2Numerous approaches were taken into account, along with the principal task’s application domains, focus (L: local; G: global), kinds of explanations collected (T: textual; V: visual), and scope (L: local; G: global) (refer Fig. 1 and Fig. 2)
Methods Considered | References | Slope | Form | Domains | ||
L | G | T | V | |||
Artificial Neural Networks (ANN) | [20, 50, 52] | + | + | Healthcare | ||
K-nearest Neighbours | [6, 46] | + | + | + | Finance | |
Deep Neural Network (DNN) | [7, 5, 58] | + | + | + | + | Criminal Sentencing |
Natural Language Processing | [60] | + | + | + | + | Judiciary |
Apparent Flow-net | [49] | + | + | Industries | ||
Attention Maps | [21, 22, 38] | + | + | Agriculture | ||
Explainable-Deep Neural Network (CNN) | [5, 7, 6] | + | + | + | Healthcare | |
Candlestick plots | [54] | + | + | + | + | Financial Market |
YOLO | [64] | + | + | Defence | ||
ROI-Net | [56] | + | + | Data Mining | ||
Deep reinforcement Learning (DRL) | [6, 8, 57] | + | + | Trading Finance | ||
Recurrent neural Network (RNN) | [7, 63] | + | + | + | Defence | |
Region-based CNN | [52] | + | + | Object Detection Aviation Sector | ||
Temporal Convolutional Neural Network (TCN) | [52] | + | Autonomous Driving | |||
Adaptive Boosting (AdaBoost) | [64] | + | + | E-commerce | ||
Convolutional Neural Network (CNN) | [52, 46] | + | + | Autonomous Driving | ||
Support Vector Machine | [9] | + | + | + | Defence | |
Logic Scoring of Preferences | [19, 20, 63] | + | + | + | Agriculture | |
Linear Discriminant Analysis (LDA) | [53] | + | + | + | E-commerce | |
Logistic Regression (LR) | [61] | + | + | + | Finance (credit scoring) | |
Linear Regression (LnR) | [64] | + | + | + | + | Sales (Forecasting sales) |
Integral Multilayer Perceptron (IMP) | [59] | + | + | Speech Recognition | ||
Bayesian Network (BN) | [51, 55] | + | + | Healthcare | ||
Gaussian Naïve Bayes classifier (GNBC) | [51] | + | + | Finance (Credit rating) | ||
Classification and Regression Tree (CART) | [34, 45, 48] | + | + | + | Agriculture |
Table 2 outlines several approaches that can be adopted for different segments of society, providing an improved understanding of the topic. In the healthcare domain, ANN have been extensively used for various tasks such as disease diagnosis, drug discovery, and personalized medicine. ANNs are particularly useful for analyzing large sets of medical data and identifying patterns that can be used to make more accurate diagnosis and treatment plans. In criminal sentencing domain, DNN have been used to predict the likelihood of reoffending and assist with sentencing decisions. DNNs are type of ANN that can handle more complex data and provide more accurate predictions. In the financial markets, candlestick plots have been used for technical analysis and predicting market trends. Candlestick plots are a type of charting technique that is used to analyze price movements in financial markets, making them an essential tool for traders and investors.
In conclusion, the use of AI techniques in different domains has shown promising results. The application of ANN in healthcare, KNN for finance, DNN for criminal sentencing, NLP for judiciary, attentional maps for agriculture, and candlestick plots for finance markets are some examples how AI has been used to improve efficiency, decision-making, and cost-effectiveness. As AI continues to evolve and become more sophisticated, we can expect to see even more exciting development in these and other domains as well.
Table 2 outlines a range of AI techniques that can be applied across several domains. Research has shown that for the healthcare industry, a significant proportion of AI techniques, around 74.48 %, were based on ANN [55]. These NN are particularly effective in analyzing large sets of medical data and identifying patterns that can be used to make more accurate diagnoses and treatment plans. Furthermore, SVM, along with RNNs contributed to approximately 53.67 % of AI techniques used in the defence domain [57]. These techniques are particularly effective in identifying potential threats and anomalies, helping to enhance security and mitigate risks. It is important to note that these figures are based on a sample of technical papers, and the actual distribution of AI techniques used in different industries may vary based on factors such as specific applications, available datasets, and industry trends. Nonetheless, these numbers provide a useful insight into the current trends in AI research and development.
Fig. 15Bar Chart representing the growth of annually published papers on various AI techniques (2017-2023)
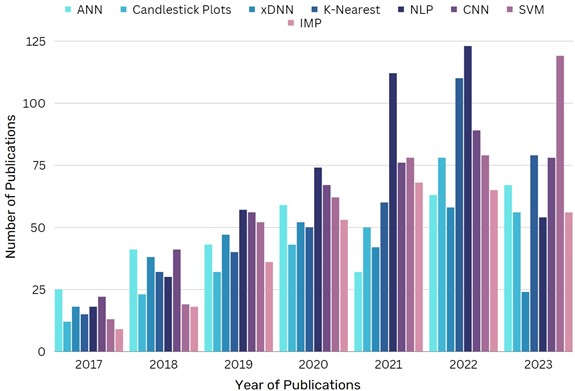
Fig. 15, the bar chart implies that the maximum publications and work done over the past few years are in the domains of Natural Language Processing. It has been observed that domains such as Judiciary, and Banking often require a text-to-speech model which is nothing but the subjugation of NLP. The judiciary domain being the adherent pillar of any nation, faces problems that can be addressed by implementing AI techniques such as Natural Language Processing and Deep Neural networks (DNNs). NLP is important because it helps resolve ambiguity in language and adds useful numeric structure to the data for many downstream applications, such as speech recognition or text analytics. The use of NLP and DNNs can indeed help address some of the challenges faced by the judiciary and banking sectors, particularly in the area of text-to speech models. By improving the accuracy and efficiency of language processing, AI techniques such as NLP can help enhance legal and financial decision-making, as well as improve customer experience found use in e-commerce applications for predicting customer behaviour and improving marketing strategies. Furthermore, the ability of NLP to resolve ambiguity in language and add useful numeric structure to data is particularly valuable in downstream applications such as speed recognition and text analytics. Overall, the use of AI techniques such as NLP and DNNs holds great potential for improving the efficiency and accuracy of various industries, including the judiciary and banking sectors. However, it is important to ensure that the use of AI is aligned with legal and ethical standards and that potential risks and limitations are carefully considered and addressed.
Fig. 16Row Chart representing the Annual global private investment in AI (2017-2023)
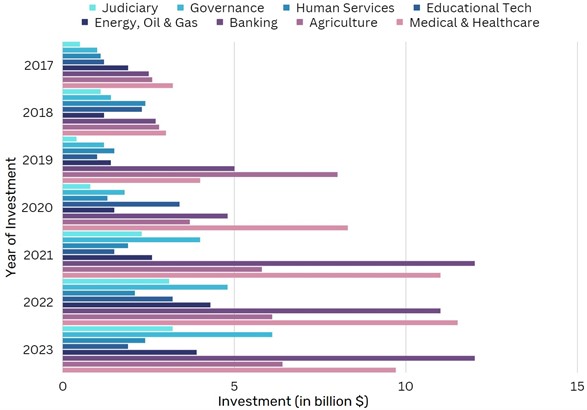
Fig. 16, the row chart implies the annual global private investment done in sectors such as Judiciary, Governance, Human Services, Educational Tech, Energy, Oil and Gas, Banking, Agriculture, and Medical-Healthcare. From the aforementioned graphical representation, it can be inferred that most of the investment done is in the Banking sector. The Agricultural sector being the most discussed province when it comes to applications of AI envisages problems such as weed, disease management, and crop health which can be tackled by adopting the classification and regression tree model. Considering the case of the Economic sector various legislation such as finance credit rating, credit scoring, and trading finance can be dealt with by individual techniques of AI such as Linear regression, Deep reinforcement learning, and Logistic regression. So, it can be concluded here that The Future of Artificial Intelligence (AI) is both exciting and uncertain. It’s impossible to predict exactly what the future of AI will look like, but one thing is certain: AI will have a profound impact on our lives. As we move into this new era of technology, it will be essential to ensure that AI is used responsibly and ethically.
Overall, the diverse range of machine learning algorithms and their applications across different domains highlights the versatility and potential of AI technology. With further research and development, we can expect to see more innovative applications of Artificial Intelligence algorithms across various industries and domains in the future.
6. Conclusions
In this article, we propose various such AI-enabled strategies, which can open doors in sectors including governance, agriculture, judiciary-legislation, and economics. Numerous AI terms including artificial neural network, neural network, deep learning, image processing, computer vision, anomaly detection, and others are used throughout the article. Overall, the use of AI technology in Governance, Judiciary, Economy and Agriculture has the potential to create significant benefits for individuals and society as a whole. These AI terms are really about models and procedures which can be used to overcome problems that are being faced by many industries nowadays by sticking rigidly with outdated traditional methods. However, the research field of seeing the world from an AI perspective is quite vast and multi-disciplinary. So, it is virtually hard to scale each and every domain using the same artificial intelligence ruler in a single article. We hope that this article can act as a jumping-off point for those who are interested in viewing the world through the lens of artificial intelligence and that by referring to it, the reader will obtain enough background to benefit from it. We also hope that the reader will find this work exciting and interesting, and if they are curious to learn more, they will seek additional research. The future of AI is exciting, and we believe that by continuing to invest in research and development, we can unlock even more potential in this rapidly growing field.
7. Future directions
As the field of AI continues to advance, future studies could integrate additional domains beyond Governance, Agriculture, Judiciary, and Economics, identifying salient features and AI-driven techniques to boost the efficacy of society even further. Sectors such as Engineering, Defence, Biomedical, Journalism, Design, Psychology, and many others can be explored in depth to fully understand the potential of AI in each domain. By providing a comprehensive overview of AI's capabilities, this article can act as a guiding torch for new researchers and pave the way for future studies that continue to explore the vast and ever-evolving world of artificial intelligence.
References
-
A. Adadi and M. Berrada, “Peeking inside the black-box: a survey on explainable artificial intelligence (XAI),” IEEE Access, Vol. 6, pp. 52138–52160, 2018, https://doi.org/10.1109/access.2018.2870052
-
A. Datta, H. Ullah, N. Tursun, T. Pornprom, S. Z. Knezevic, and B. S. Chauhan, “Managing weeds using crop competition in soybean [Glycine max (L.) Merr.],” Crop Protection, Vol. 95, pp. 60–68, May 2017, https://doi.org/10.1016/j.cropro.2016.09.005
-
S. A. Al-Ghamdi, J. Khabti, and H. S. Al-Khalifa, “Exploring NLP web APIs for building Arabic systems,” in Twelfth International Conference on Digital Information Management (ICDIM), Sep. 2017, https://doi.org/10.1109/icdim.2017.8244649
-
P. Angelov and E. Soares, “Towards explainable deep neural networks (xDNN),” Neural Networks, Vol. 130, pp. 185–194, Oct. 2020, https://doi.org/10.1016/j.neunet.2020.07.010
-
A. N. Rao, S. Wani, and J. K. Ladha, “Weed management research in India an analysis of past and outlook for future,” DWR Souvenir, Publication 18, 2014.
-
G. S. Atsalakis and K. P. Valavanis, “Surveying stock market forecasting techniques – Part II: Soft computing methods,” Expert Systems with Applications, Vol. 36, No. 3, pp. 5932–5941, Apr. 2009, https://doi.org/10.1016/j.eswa.2008.07.006
-
A. Bahrammirzaee, “A comparative survey of artificial intelligence applications in finance: artificial neural networks, expert system and hybrid intelligent systems,” Neural Computing and Applications, Vol. 19, No. 8, pp. 1165–1195, Nov. 2010, https://doi.org/10.1007/s00521-010-0362-z
-
B. Ji, Y. Sun, S. Yang, and J. Wan, “Artificial neural networks for rice yield prediction in mountainous regions,” The Journal of Agricultural Science, Vol. 145, No. 3, pp. 249–261, Jun. 2007, https://doi.org/10.1017/s0021859606006691
-
K. Barefoot et al., “Defining and measuring the digital economy,” US Department of Commerce Bureau of Economic Analysis, Washington, 2018.
-
T. J. Buocz, “Artificial Intelligence in Court Legitimacy Problems of AI Assistance in the Judiciary,” Retskraft-Copenhagen Journal of Legal Studies, Vol. 2, No. 1, pp. 41–59, 2018.
-
C. R. de Kimpe and J.-L. Morel, “Urban soil management: a growing concern,” Soil Science, Vol. 165, No. 1, pp. 31–40, Jan. 2000, https://doi.org/10.1097/00010694-200001000-00005
-
B. Chen, Y. Li, S. Zhang, H. Lian, and T. He, “A deep learning method for judicial decision support,” in IEEE 19th International Conference on Software Quality, Reliability and Security Companion (QRS-C), Jul. 2019, https://doi.org/10.1109/qrs-c.2019.00040
-
M. Chen, U. Challita, W. Saad, C. Yin, and M. Debbah, “Artificial neural networks-based machine learning for wireless networks: a tutorial,” IEEE Communications Surveys and Tutorials, Vol. 21, No. 4, pp. 3039–3071, 2019, https://doi.org/10.1109/comst.2019.2926625
-
K. R. Chowdhary, “Natural language processing,” in Fundamentals of Artificial Intelligence, New Delhi: Springer India, 2020, pp. 603–649, https://doi.org/10.1007/978-81-322-3972-7_19
-
S. Dragićević and K. Hatch, “Urban geosimulations with the logic scoring of preference method for agent-based decision-making,” Habitat International, Vol. 72, pp. 3–17, Feb. 2018, https://doi.org/10.1016/j.habitatint.2017.09.006
-
R. Sharma, “Artificial intelligence in agriculture: a review,” in 5th International Conference on Intelligent Computing and Control Systems (ICICCS), May 2021, https://doi.org/10.1109/iciccs51141.2021.9432187
-
M. Dymitruk, “Ethical artificial intelligence in judiciary,” in 22nd International Legal Informatics Symposium IRIS, 2019.
-
E. J. van Henten et al., “An autonomous robot for harvesting cucumbers in greenhouses,” Autonomous Robots, Vol. 13, No. 3, pp. 241–258, 2002, https://doi.org/10.1023/a:1020568125418
-
E. M. López, M. García, M. Schuhmacher, and J. L. Domingo, “A fuzzy expert system for soil characterization,” Environment International, Vol. 34, No. 7, pp. 950–958, Oct. 2008, https://doi.org/10.1016/j.envint.2008.02.005
-
F. López-Granados, “Weed detection for site-specific weed management: mapping and real-time approaches,” Weed Research, Vol. 51, No. 1, pp. 1–11, Feb. 2011, https://doi.org/10.1111/j.1365-3180.2010.00829.x
-
M.-Y. Chen, A. K. Sangaiah, T.-H. Chen, E. D. Lughofer, and E. Egrioglu, “Deep learning for financial engineering,” Computational Economics, Vol. 59, No. 4, pp. 1277–1281, Apr. 2022, https://doi.org/10.1007/s10614-022-10260-8
-
D. Goularas and S. Kamis, “Evaluation of deep learning techniques in sentiment analysis from twitter data,” in 2019 International Conference on Deep Learning and Machine Learning in Emerging Applications (Deep-ML), Aug. 2019, https://doi.org/10.1109/deep-ml.2019.00011
-
J. Han, J. Tao, and C. Wang, “FlowNet: a deep learning framework for clustering and selection of streamlines and stream surfaces,” IEEE Transactions on Visualization and Computer Graphics, pp. 1–1, 2019, https://doi.org/10.1109/tvcg.2018.2880207
-
J. L. Hatfield, M. Cryder, and B. Basso, “Remote sensing: advancing the science and the applications to transform agriculture,” IT Professional, Vol. 22, No. 3, pp. 42–45, May 2020, https://doi.org/10.1109/mitp.2020.2986102
-
Z. Huang, H. Chen, C.-J. Hsu, W.-H. Chen, and S. Wu, “Credit rating analysis with support vector machines and neural networks: a market comparative study,” Decision Support Systems, Vol. 37, No. 4, pp. 543–558, Sep. 2004, https://doi.org/10.1016/s0167-9236(03)00086-1
-
C. Huang, Z. Zhang, B. Mao, and X. Yao, “An overview of artificial intelligence ethics,” IEEE Transactions on Artificial Intelligence, Vol. 4, No. 4, pp. 799–819, Aug. 2023, https://doi.org/10.1109/tai.2022.3194503
-
R. Huang, J. Pedoeem, and C. Chen, “YOLO-LITE: a real-time object detection algorithm optimized for Non-GPU computers,” in 2018 IEEE International Conference on Big Data (Big Data), Dec. 2018, https://doi.org/10.1109/bigdata.2018.8621865
-
Shaan Shah. “Identifying candlestick patterns using deep learning.” 2020, https://towardsdatascience.com/identifying-candlestick-patterns-using-deep-learning-b7d706726874.
-
S. Fahad et al., “Weed growth and crop yield loss in wheat as influenced by row spacing and weed emergence times,” Crop Protection, Vol. 71, pp. 101–108, May 2015, https://doi.org/10.1016/j.cropro.2015.02.005
-
Shuiwang Ji and Jieping Ye, “Generalized linear discriminant analysis: a unified framework and efficient model selection,” IEEE Transactions on Neural Networks, Vol. 19, No. 10, pp. 1768–1782, Oct. 2008, https://doi.org/10.1109/tnn.2008.2002078
-
M. J. Donoghue, C. D. Bell, and J. Li, “Phylogenetic patterns in northern hemisphere plant geography,” International Journal of Plant Sciences, Vol. 162, No. S6, pp. S41–S52, Nov. 2001, https://doi.org/10.1086/323278
-
K. Neil Harker, “Survey of yield losses due to weeds in central Alberta,” Canadian Journal of Plant Science, Vol. 81, No. 2, pp. 339–342, Apr. 2001, https://doi.org/10.4141/p00-102
-
M. Khan and N. Haq, “Wheat crop yield loss assessment due to weeds,” Sarhad Journal of Agriculture, 2002.
-
M. Pagliai, N. Vignozzi, and S. Pellegrini, “Soil structure and the effect of management practices,” Soil and Tillage Research, Vol. 79, No. 2, pp. 131–143, Dec. 2004, https://doi.org/10.1016/j.still.2004.07.002
-
M. S. Moran, Y. Inoue, and E. M. Barnes, “Opportunities and limitations for image-based remote sensing in precision crop management,” Remote Sensing of Environment, Vol. 61, No. 3, pp. 319–346, Sep. 1997, https://doi.org/10.1016/s0034-4257(97)00045-x
-
A. B. Malali and S. Gopalakrishnan, “Application of artificial intelligence and its powered technologies in the indian banking and financial industry: An overview.,” IOSR Journal of Humanities And Social Science (IOSR-JHSS), Vol. 25, No. 4, pp. 55–60, 2020, https://doi.org/10.9790/0837-2504065560
-
T. Mikolov, M. Karafiát, L. Burget, J. Černocký, and S. Khudanpur, “Recurrent neural network based language model,” Interspeech 2010, Sep. 2010, https://doi.org/10.21437/interspeech.2010-343
-
M. Mishra and M. Srivastava, “A view of artificial neural network,” in 2014 International Conference on Advances in Engineering and Technology Research (ICAETR), Aug. 2014, https://doi.org/10.1109/icaetr.2014.7012785
-
T. T. Nguyen and V. J. Reddi, “Deep reinforcement learning for cyber security,” IEEE Transactions on Neural Networks and Learning Systems, pp. 1–17, 2021, https://doi.org/10.1109/tnnls.2021.3121870
-
Nibedita Saha. “Rise of AI in the Indian economy.”. https://indiaai.gov.in/article/rise-of-ai-in-the-indian-economy (accessed 2020).
-
P. Debaeke and A. Aboudrare, “Adaptation of crop management to water-limited environments,” European Journal of Agronomy, Vol. 21, No. 4, pp. 433–446, Dec. 2004, https://doi.org/10.1016/j.eja.2004.07.006
-
S. Pathak, I. Mishra, and A. Swetapadma, “An Assessment of decision tree based classification and regression algorithms,” in 2018 3rd International Conference on Inventive Computation Technologies (ICICT), Nov. 2018, https://doi.org/10.1109/icict43934.2018.9034296
-
D. P. C. Peters, H. M. Savoy, G. A. Ramirez, and H. Huang, “AI recommender system with ML for agricultural research,” IT Professional, Vol. 22, No. 3, pp. 30–32, May 2020, https://doi.org/10.1109/mitp.2020.2986125
-
R. Posevkin and I. Bessmertny, “Translation of natural language queries to structured data sources,” in 2015 9th International Conference on Application of Information and Communication Technologies (AICT), Oct. 2015, https://doi.org/10.1109/icaict.2015.7338516
-
R. Gerhards and S. Christensen, “Real-time weed detection, decision making and patch spraying in maize, sugarbeet, winter wheat and winter barley,” Weed Research, Vol. 43, No. 6, pp. 385–392, Dec. 2003, https://doi.org/10.1046/j.1365-3180.2003.00349.x
-
“5 Real-world examples of logistic regression application,” https://activewizards.com/blog/5-real-world-examples-of-logistic-regression-application
-
Reiling and A. D. (Dory), “Courts and artificial intelligence,” International Journal for Court Administration, Vol. 11, No. 2, Aug. 2020, https://doi.org/10.36745/ijca.343
-
Kara Longo Korte. “How to measure the ROI for artificial intelligence,” 2020, https://www.spiceworks.com/tech/artificial-intelligence/guest-article/how-to-measure-the-roi-for-artificial-intelligence/
-
S. J. Russell, Artificial Intelligence a Modern Approach. Pearson Education, Inc., 2010.
-
J. Behmann, A.-K. Mahlein, T. Rumpf, C. Römer, and L. Plümer, “A review of advanced machine learning methods for the detection of biotic stress in precision crop protection,” Precision Agriculture, Vol. 16, No. 3, pp. 239–260, Jun. 2015, https://doi.org/10.1007/s11119-014-9372-7
-
S. Kolhe, Raj Kamal, H. S. Saini, and G. K. Gupta, “An intelligent multimedia interface for fuzzy-logic based inference in crops,” Expert Systems with Applications, Vol. 38, No. 12, pp. 14592–14601, Nov. 2011, https://doi.org/10.1016/j.eswa.2011.05.023
-
S. C. Kumain, K. Kumain, and P. Chaudhary, “AI impact on various domain: an overview,” International Journal of Management, Vol. 11, No. 10, Dec. 2020.
-
H. Shakouri, G. R. Nadimi, and F. Ghaderi, “Fuzzy linear regression models with absolute errors and optimum uncertainty,” in 2007 IEEE International Conference on Industrial Engineering and Engineering Management, Dec. 2007, https://doi.org/10.1109/ieem.2007.4419325
-
W.-T. Tsai, X. Sun, and J. Balasooriya, “Service-oriented cloud computing architecture,” in 2010 Seventh International Conference on Information Technology: New Generations, 2010, https://doi.org/10.1109/itng.2010.214
-
C.-F. Tsai, “Feature selection in bankruptcy prediction,” Knowledge-Based Systems, Vol. 22, No. 2, pp. 120–127, Mar. 2009, https://doi.org/10.1016/j.knosys.2008.08.002
-
D. Valle-Cruz, E. Alejandro Ruvalcaba-Gomez, R. Sandoval-Almazan, and J. Ignacio Criado, “A review of artificial intelligence in government and its potential from a public policy perspective,” in 20th Annual International Conference on Digital Government Research, Jun. 2019, https://doi.org/10.1145/3325112.3325242
-
“Facts About Weeds.” Weed Science Society of America, https://wssa.net/wp-content/uploads/wssa-fact-sheetfinal.pdf
-
L. Zhu, “Application of Naive Bayesian classifier based on gaussian distribution in plant recognition,” in 2021 IEEE 12th International Conference on Software Engineering and Service Science (ICSESS), pp. 135–139, Aug. 2021, https://doi.org/10.1109/icsess52187.2021.9522331
-
K. C. Desouza, G. S. Dawson, and D. Chenok, “Designing, developing, and deploying artificial intelligence systems: Lessons from and for the public sector,” Business Horizons, Vol. 63, No. 2, pp. 205–213, Mar. 2020, https://doi.org/10.1016/j.bushor.2019.11.004
-
Laura Galindo, Karine Perset, and Francesca Sheeka, “An overview of national AI strategies and policies,” Going Digital Toolkit Notes, Aug. 2021, https://doi.org/10.1787/c05140d9-en
-
Kummitha and Rama Krishna Reddy, “Smart technologies for fighting pandemics: The techno – and human – driven approaches in controlling the virus transmission,” Government Information Quarterly, Vol. 37, No. 3, p. 101481, Jul. 2020, https://doi.org/10.1016/j.giq.2020.101481
-
“Recommendation on the ethics of artificial intelligence,” Unesco, 2021.
About this article
The authors have not disclosed any funding.
The datasets generated during and/or analyzed during the current study are available from the corresponding author on reasonable request.
Rohan Kishor: conceptualization, writing-original draft preparation, reviewing and editing. Bhumeshwar Patle: conceptualization, writing-original draft preparation, reviewing and editing. Praveen Bhojane: writing-original draft preparation.
The authors declare that they have no conflict of interest.