Abstract
In order to excavate the relationship between the different features of the vibration signal, and to provide more useful information for the fault diagnosis of rolling bearings, this paper developed a new method of fault diagnosis-health baseline method and introduced the technological process of this method in detail. Through the case study, a health baseline based on two kinds of linear models was constructed. After testing, this method can distinguish the normal state of the rolling bearing, the external ring fault and the rolling element fault, which indicates that the method was feasible and effective for the fault diagnosis of the rolling bearing.
1. Introduction
Rolling bearings are the key components of rotating machinery and equipment, which play a pivotal role in many industrial areas, the health of rolling bearings is essential for the performance and reliability of mechanical equipment [1]. Therefore, the fault diagnosis of rolling bearings in industrial production has a very important significance.
In recent years, the pattern recognition method has gradually become the mainstream method of fault diagnosis and has attracted attentions of many scholars [2]. The method does not need to establish the mathematical model of the system, but it is a data-driven fault diagnosis method based on the vibration data of the bearing collected by the sensor to recognize its working state. In a sense, the fault diagnosis of rolling bearings is the process of identifying and classifying its characteristic patterns. In the past studies, different scholars have used a lot of vibration signal processing methods for this problem, including: discrete/random separation (DRS) method, extreme learning machine, quaternion singular spectrum analysis and so forth [3-5]. Most of these methods are effective on a particular problem, but they usually use only one single feature or a few features of the vibration signal, which might ignore the relationship between the different characteristics of the signal and resulted in missing information.
In the last few years, with the rise of big data technology, mining key information through data association is becoming an effective approach to solve practical problems and locate targets [6]. In the industrial field based on the internet of things, the faulty machine can be recognized by collecting the sensor data in time and establishing the correlation relation between the different sensor state curve changes to distinguish the abnormal points [7]. In social networking applications, there have been scholars who have put forward an association that could be identified from different social platform data to identify the same identity users [8].
The information mentioned provides a new idea for the fault diagnosis of rolling bearings. We can find out the relationship between the normal state and the rolling bearing vibration signals in different failure modes and then reveal the difference of these relationships as the benchmark for fault diagnosis. Based on this idea, this paper develops a health baseline method to achieve the signal classification and fault diagnosis by analyzing different relationships between the features and building the health baseline. This paper was structured as follows: The second section illustrated the theory and main processes of building the health baseline; the third section detailed the application case of the diagnosis of rolling bearings based on the health baseline method and demonstrate the analysis and evaluation of its effect; the fourth section discussed the health baseline method; the fifth section made the conclusion.
2. Methodology
The purpose of building a health baseline was to distinguish the working state of the object. Its essence can be a quantitative formula or a description of tendency. At the same time, it was possible to apply one parameter or a variety of parameters to build the health baseline. For vibration signals, the flow path of health baseline construction was shown in Fig. 1, and it can be divided into three parts:
Firstly, the same features were extracted from the normal data and the fault data whose fault type was known. The time domain features, frequency domain features or other features can represent its signal characteristics can be extracted. The more the feature was extracted, the more useful information could be excavated, which was favorable to diagnosis.
Secondly, we analyzed the parameters climate of the features extracted from the signals. Theoretically, the parameters climate of normal data and the fault data parameters climate must be different, which was the root cause that the health baseline can be built. According to the parameters climate of normal data, the health baseline in normal condition could be built, and the same for the fault data. There can be a variety of methods to analyze the relationship between the parameters, such as regression algorithms, SPSS analysis or Apriori algorithm, FP-Growth algorithm which were commonly used in big data area [9-11].
Finally, the baseline must be verified to ensure the accuracy to fault diagnosis.
Fig. 1The procedure of the proposed methodology
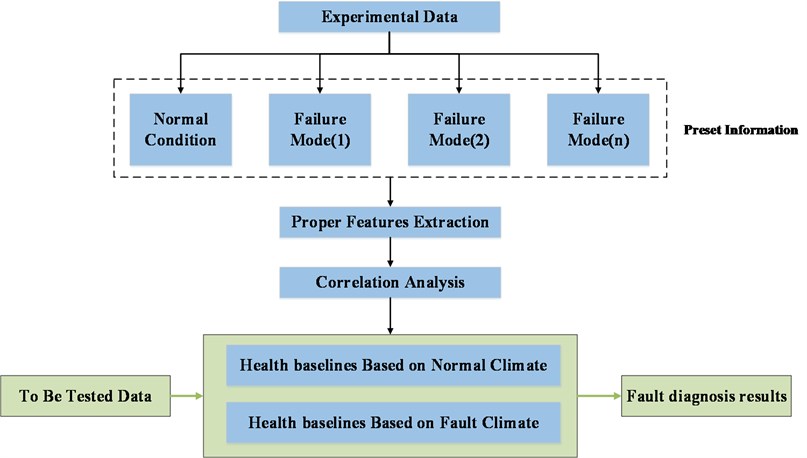
3. Case study
In this paper, the data was collected by using rotating test bench. The conditions of rolling bearings included normal mode, external ring failure, rolling element failure, and the revolving speed was set to 2400 rpm with no external load. The data collection point for the bearing was just above the bearing, that was, 12 o’clock direction. The vibration sensor was fixed in magnetic way. Then the vibration data was collected by using vibration sensor and data acquisition equipment from the rotating test bench in real-time, and the length of each acquisition was 1000 points.
For every vibration signal, the energy, skewness factor, kurtosis factor, root mean square value (R. M. S.) and the average absolute value were extracted. In order to obtain the features information deep-going, we set 25 points as a group according to the time series so that each collected data was divided into 40 groups. The normal data eigenvalues were shown in Fig. 2.
Fig. 2The features value of normal data
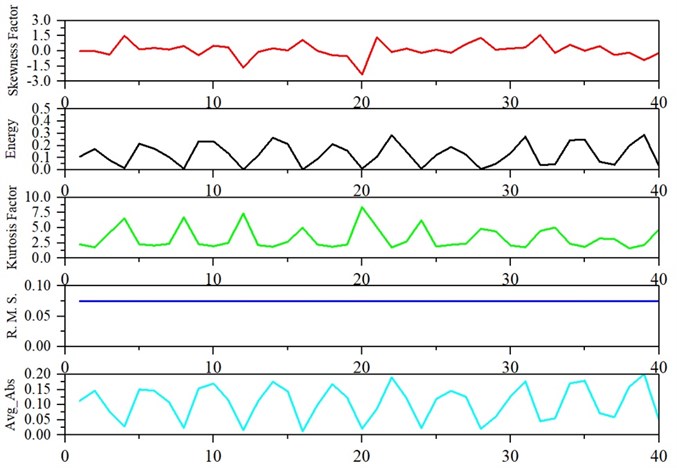
Table 1The fitting coefficients of linear models
Fitting coefficient | Avg_Abs | Energy | Kurtosis factor | Constant |
Normal | 1 | 0.473 | –0.009 | 0.074 |
External ring fault | 1 | 15.756 | –0.091 | 1.496 |
Rolling element fault | 1 | 0.465 | –0.003 | 0.052 |
By using SPSS software, it was found that the energy and kurtosis factors of the vibration signals showed a significant linear correlation with the average absolute value in each condition, and the fitting degree of each model was more than 95 %. But the linear model in different states was not same. In detail, the average absolute value was set as the dependent variable, the energy and the kurtosis factor were set as the independent variables. The linear model coefficients in the three states were shown in Table 1, and the fitting diagrams were shown in Fig. 3.
As can be seen from Fig. 3, the external ring fault was significantly different from the other two states, and the normal state and the rolling body failure were obviously different but still intersecting, so further analysis was needed.
After comparing the curves, it was found that the three states show a quantitative relationship with the eigenvalues of R. M. S. (denoted as ), which were:
After comparing the eigenvalues R. M. S. of the intersections in Fig. 3, it can be distinguished by the signal as a normal state signal or a rolling element fault signal.
In summary, the linear model plus the Eqs. (1) (2) constituted a healthy baseline for rolling bearings. For the constructed health baseline, the accuracy of the fault diagnosis was 100 % by using three kinds of signal tests randomly. It was proved that the method was effective for fault diagnosis of rolling bearing.
Fig. 3The fitting diagrams under different state
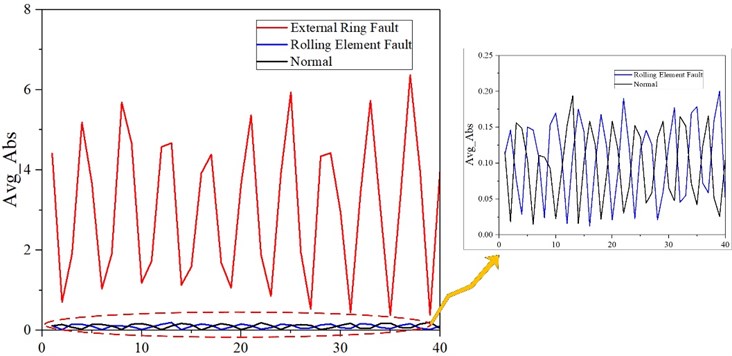
4. Discussion
(1) The health baseline approach used in this paper was based on the form of equation, however, there could be more forms to construct the health baseline. It can be a qualitative construction and also can be a combination of qualitative and quantitative construction, and the criterion for determining whether a health baseline is suitable for fault diagnosis is to identify well and classify the signal to an acceptable Level.
(2) For a variety of the health baselines can be built from different angles in the same system, we can achieve a quick diagnostic. For example, in this paper, if we only need to estimate whether there is a failure in bearing, we only need to input signal-related feature parameters into the normal state of the model to test whether it meets the health baseline.
(3) There are both similarities and differences between health baseline method and other diagnosis methods. First, the data-based association analysis is a purely numerical analysis, and whether the relationship has a physical meaning is uncertain, if the association has a certain physical meaning, it is to some extent equivalent to obtain the analytic model of the system, and fault diagnosis based on the analytic model is also an important direction of fault diagnosis; Secondly, analyzing the normal signal to get a health baseline is similar to the observer method commonly used in the control system, but the description of the failure is different. In a word, the health baseline is a systematic approach that is considered from multiple perspectives.
(4) The advantage of the health baseline is that once the health baseline is cured, then subsequent diagnostics can be targeted and the whole process is rapid, which is very useful for systems with known failure modes and fewer failure modes. The disadvantage of this approach is that it is time-consuming and difficult to build a health baseline that can effectively differentiate each failure mode for systems with known failure and more failure modes. For systems with an unknown failure mode, the problem is that we cannot evaluate the effect of the built health baseline.
5. Conclusions
In this paper, a new method of fault diagnosis was proposed. By extracting the different features of the vibration signals of the rolling bearing and mining the relationship among them, a health baseline was built based on two kinds linear models. It is proved by the experiments that the method can effectively distinguish the state of signals and it had high dependability.
References
-
Li B., Chow M. Y., Tipsuwan Y., et al. Neural-network-based motor rolling bearing fault diagnosis. IEEE Transactions on Industrial Electronics, Vol. 47, Issue 5, 2000, p. 1060-1069.
-
Shuang L. Fault pattern recognition of rolling bearing based on singularity value decomposition and support vector machine. Transactions of the Chinese Society of Agricultural Engineering, 2007.
-
Randall R. B., Antoni J. Rolling element bearing diagnostics – a tutorial. Mechanical Systems and Signal Processing, Vol. 25, Issue 2, 2011, p. 485-520.
-
Tian Y., Ma J., Lu C., et al. Rolling bearing fault diagnosis under variable conditions using LMD-SVD and extreme learning machine. Mechanism and Machine Theory, Vol. 90, 2015, p. 175-186.
-
Yi C., Lu Y., Dang Z., et al. Quaternion singular spectrum analysis using convex optimization and its application to fault diagnosis of rolling bearing. Measurement, Vol. 103, 2017, p. 321-332.
-
Hu H., Wen Y., Chua T. S., et al. Toward scalable systems for big data analytics: a technology tutorial. IEEE Access, Vol. 2, Issue 1, 2017, p. 652-687.
-
Ziegel E. R. Fault Detection and Diagnosis in Industrial Systems. Advanced Textbooks in Control and Signal Processing, Vol. 12, Issue 3, 2002, p. 453-454.
-
Mutawa N. A., Baggili I., Marrington A. Forensic analysis of social networking applications on mobile devices. Digital Investigation, Vol. 9, Issue 15, 2012, p. 24-33.
-
Trawiński B., Smętek M., Telec Z., et al. Nonparametric statistical analysis for multiple comparison of machine learning regression algorithms. International Journal of Applied Mathematics and Computer Science, Vol. 22, Issue 4, 2012, p. 449-471.
-
Preacher K. J., Hayes A. F. SPSS and SAS procedures for estimating indirect effects in simple mediation models. Behavior Research Methods Instruments and Computers, Vol. 36, Issue 4, 2004, p. 717-731.
-
Aflori C., Craus M. Grid implementation of the apriori algorithm. Advances in Engineering Software, Vol. 38, Issue 5, 2007, p. 295-300.
About this article
The authors declare that there is no any potential conflict of interests in the research. This study is supported by the Fundamental Research Funds for the Central Universities (Grant Nos. YWF-17-BJ-J-42 and YWF-16-BJ-J-18) and the National Natural Science Foundation of China (Grant Nos. 51605014 and 51575021), as well as the Technology Foundation Program of National Defense (Grant No. Z132013B002).