Abstract
Difficult-to-machine materials, e.g., Titanium alloys, are highly applicable in diverse industries that yield strength and wear resistance. However, they prove difficult to machine due to high vibration, leading to high cutting forces during the machining process. This vibration occurs from chip discontinuity and thereby leads to high friction between the cutting tool and workpiece. In order to minimize these challenges, lubricants are employed in machining operations to reduce frictional and other unnecessary cutting forces and improve surface finish. This research focuses on studying the nano-lubricant effects in reducing cutting forces in the machining of TI-6AL-4V-ELI alloy. Also, carry out a comparative study of dry, mineral oil, and TiO2 nano-lubricant during face-milling machining for optimal performance. Additionally, the study develops a predictive mathematical model for cutting force using a Taguchi L9 orthogonal array. A two-step approach was employed to develop the nano-lubricant before the machining process. The dynamometer is used to collect the cutting force data at the end of each sample. The Results show that the lubrication conditions play a significant role in the reduction of cutting forces. The mineral oil-based-TiO2 nano-lubricant reduces the cutting force by 19 % compared with the mineral oil during the machining of TI-6AL-4V-ELI alloy. Furthermore, the optimal parameters to reduce cutting forces during face milling of TI-6AL-4V-ELI alloy are cutting speed at 3000 rpm, 200 mm/min feed rate, 0.3 mm depth of cut to obtain the minimum cutting force 30 (N). This study concludes that the application of TiO2 nanoparticles in mineral oil significantly improves the thermal and mechanical properties, which leads to a reduction of cutting force.
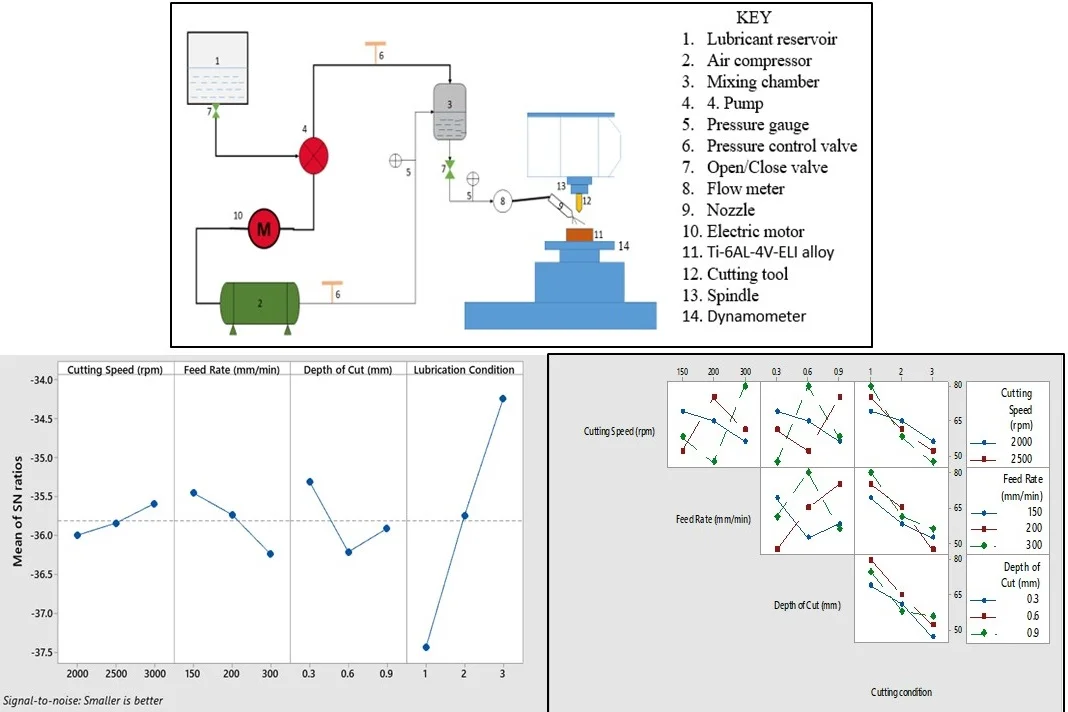
Highlights
- The influence of the TiO2 nanoparticles on the mineral oil mechanical properties shows a significant difference during the machining of TI-6AL-4V-ELI alloy on the cutting force
- The optimal machining parameters during machining TI-6AL-4V-ELI alloy was achieved
- The study developed a predictive mathematical model for cutting force using a Taguchi L9 orthogonal array and carried out an interaction study of parameters
1. Introduction
Complex to cut metals are metals produced for the sole purpose of withstanding extreme conditions and applications [1]. Due to this essential characteristic needed from these metals, the manufacturers increase the hardness, corrosion resistance and reduce thermally conductive to make the metals more preferable than other metals, which has led to the difficulty in machining them during the manufacturing process. Hence they are classified as difficult-to-cut metals [2]. Due to their impressive chemical and mechanical properties, difficult-to-machine materials such as Titanium alloys are highly applicable in the automotive and aerospace industries due to their high strength-to-weight ratio, durability, creep, corrosion resistivity, and wear resistance. According to Hegab et al. [3], the grades of Titanium alloy and their applications are:
1) Grades 1: This is titanium's most ductile and, therefore, the softest category. It possesses high corrosion
2) Grade 5: Ti6Al4V. The most commonly used grade of titanium. Stronger than Grade 1-4 Titanium. It possesses the same grade properties as grades 1-4, except 60 % less thermal conductivity when compared.
3) Grade 6: Ti-5Al-2.5Sn. It is used in airplanes and jet engines and has good weld-ability, good strength, and stability at high temperatures.
4) Grade 7/7H: Contains 0.12-0.23 % palladium and possesses enhanced crevice corrosion resistance.
5) Grade 9: Contains 3 % aluminum, 2.5 % vanadium, Good weld-ability, High strength, used in aircraft tubing.
6) Grade 11: 0.12 %-0.25 % palladium. Enhanced corrosion resistance.
7) Grade 16/16H/17: 0.4-0.08 % palladium and possesses enhanced corrosion resistance.
8) Grade 18: Contains 3 % of Aluminium, 2.5 % of vanadium. This grade possesses similar mechanical properties as grade 9, with enhanced corrosion resistance as grade 16.
9) Grade 23: Ti6Al-4V-ELI. It contains 6 % aluminum, 4 % vanadium, 0.13 % oxygen (max), good ductility, and tough so it can resist fracture, but the strength is reduced. Most commonly used for medical implants.
10) Grade 38: 2.5 % vanadium, 4 % Aluminium, 1.5 % iron. It is used for armor plating, and it possesses the same properties as grade 5, good cold workability similar to grade 9.
Several problems have been raised during machining Titanium alloy [4]. Furthermore, this study will apply nano-lubricant during the machining process to reduce these problems ranging from short tool life, high heat generated at cutting zone, frictional, and cutting forces. This nano-lubricant will further improve surface finish lubricants and reduce cutting force. Over the years’ improvements and continuous research relating to machining operations have given machinists the ability to improve lubricants' quality and performance using nanoparticles [5-6]. Applying nanoparticles to base oil improves the lubricant oil characteristics, improving wear resistance and reducing friction. According to Gaurav et al. [7] and Khalil et al. [8], nanoparticles improve load-carrying capacity and reduce cutting forces. Experiment on a Nickel-Titanium alloy to see the effect of nano-lubricants on the alloy is why studying cutting forces and tool wear. The results show that the nano-lubricant successfully reduced cutting forces by 6-10 % and tool wear by 4.2-34.5 %. The nanoparticle used in this experiment was Al2O3, with soluble cutting oil (Sol-Cut) as the base oil. Hegab et al. [9] carried out a study with promising results on the best way to preserve the process for parameters when cutting 718 Inconel using nano-lubricants minimum quantity lubrication technique. The nanoparticles used are MWCNTs and Al2O3. Also, Sarhan et al. [10] work on the reduction of power in the milling process using the SiO2 nano-lubrication system show that:
1) SiO2 nanoparticles dispersed in mineral oil improve machining process performance by reducing COF and cutting forces.
2) Smaller specific energy and the power required in machining processes are obtained using nano-lubrication systems compared to ordinary lubrication systems.
3) With the nano-lubrication system's application, the increase of cutting forces is reduced compared to ordinary lubrication systems.
Even without the use of base oils, nanoparticles can still be applied dry. A review of Ghaednia and Jackson's [11] work on the dry application of CuO shows that these particles can reduce friction and wear even with lubricants’ absence. A review carried out by Zareh-Desari, and Davoodi [12] shows that SiO2 and CuO nanoparticles dispersed in vegetable oil can reduce friction by 21-31 %. Hence power consumption and cutting forces are substantially reduced. The performance of nano-lubricants was concluded to be superior as compared to conventional machining fluid. The addition of nano-graphene to vegetables produced better surface quality, central tool wear, and flank tool wear juxtaposing to just vegetable oil. This study is in line with the opinion made by [13-14]. Rahman et al. [15] research was carried out on nanofluids to improve base fluids' lubricating effect in turning biomedical grade titanium. The author's results show that the lowest Ra (surface roughness) value recorded, i.e. (0.248 µm) for the addition of 0.5 vol%-Al2O3-CAN nanofluid, 73.1 % less than that recorded for dry cutting.
Furthermore, the lowest temperature was recorded at 875oC when 0.5 %-MoS2 was used as the nanoparticle and the most massive Chip thickness (R.C.) at 0.9238, implying a substantial decrease in cutting forces COF.
According to Anand et al. [16], the application of TiO2 and Graphine nanoparticles in rice bran oil improves the tribological properties of the base fluid. The study also shows that the tool wear rate was reduced due to the application of the nanoparticles. Li et al. [17] performed a quantitative analysis of the lubricating and cooling effects of graphene oxide nano-fluids in the machining of titanium alloy (Ti6Al4V). The results showed that both the frictional force and friction coefficient on the flank face were significantly moderated with graphene oxide nano-sheets. The smaller normal pressure made the nano-sheets easier to make into the tool/workpiece interface, minimizing the tool/workpiece abrasion.
Minimizing cutting forces is one of the essential machining of Titanium alloy issues because less cutting forces would mean cheaper machining costs. High cutting forces are a challenge in machining titanium because of its hardness [18-19]. There is a relationship between cutting force and tool wear rate, as high cutting force will lead to a high cutting tool wear rate [20]. Today, lubricants are used in the reduction of these forces. Research and development in this area have improved these lubricants’ properties with nanoparticles’ introduction [21]. Although this is undoubtedly a fantastic engineering feat, nano lubrication still leaves many unchartered-water to how relevant it is in manufacturing processes. Not many tests and research has been carried out on nano-lubricants, causing a deficit in the engineering bulk of knowledge. This research is keen on finding out to what extent these improved lubricants reduce the cutting forces in the machining of titanium alloys. The case study nanoparticles are TiO2 nanoparticles, hence adding to the bulk of knowledge acquired subject matter. Industries are searching for a sustainable way of reducing costs by cutting down unnecessary wastes while preserving the quality and quantity of manufactured goods. High cutting forces prevent the excellent quality finish of machined components and cause premature wear and damage to tools hence running companies at a loss during the manufacturing process.
Furthermore, there is a need to study eco-friendly lubricants and nano-lubricant effects on the cutting force during the machining of the difficult-to-cut materials for the sustainable manufacturing process. Therefore, this research will carry out a comparative analysis on the three machining conditions such as dry machining, mineral oil, and mineral oil-based TiO2 nano-lubricant effect on cutting force reduction during machining of Ti-6AL-4V-ELI alloy, which has not been studied. In aeronautics, aerospace, and medicine, where titanium is a popular metal, a high-quality finish is essential; hence this study is relevant to meeting the need. In order to achieve this, the following objectives are:
1) Analyses the effects of synthesized TiO2 nano-lubricant on the Ti-6AL-4V-ELI alloy during face-milling machining and also carry out the comparative analysis of the dry and mineral oil with the machining parameters.
2) Develop a predictive mathematical model for cutting force, using Taguchi design.
The study employs an L9 Taguchi design with four factors at three levels of experiments, and the factors are cutting speed, feed rate, depth-of-cut, and lubrication conditions on the response (cutting force).
2. Materials and methods
The XM1060 CNC machine with the power supply of 400V-50HZ-3PH, spindle speed max of 10000 rpm, rated power of 21 KW, and weight 6980 Kg was used to carry out the face milling operation. This machine is located in the Mechanical Engineering Department of Covenant University, Ota, Nigeria. The specification of the cutting tool’s chemical composition is given in Table 1. The material workpiece considered for this experiment is a rectangular Ti-6AL-4V-ELI alloy (Grade 23) Titanium block of 2000 mm×50 mm×5 mm, procured from a local scrap yard in Lagos. The chemical composition, mechanical and physical properties are given in Tables 2 to 4.
The selected nanoparticle employed for this experiment are:
Titanium dioxide (TiO2): These nanoparticles possess diameters less than a hundred nanometres, i.e., 15 nm. One everyday use for these nanoparticles is in the production of sunscreen. The significance of the TiO2 nanoparticle implemented in this study is its high rate of corrosion resistance and the ability to increase the cooling rate of the base oil. The base oil for this experiment is white mineral oil. White mineral oil is accredited for being odorless, colorless, transparent, and possessing a non-fluorescent hydrocarbon blend. It finds its use in the food industry and in dissolving nanoparticles.
Table 1Chemical composition of cemented milling carbide insert tool
Elements | Co | TAC | NBC | WC |
Weight % | 9.1 | 1.23 | 0.30 | 89.37 |
Table 2Chemical composition of Ti-6AL-4V-ELI alloy
Elements | Ti | Al | V | O2 |
Weight % | 89.69 % | 6 % | 4 % | 0.13 % |
Table 3Mechanical properties of Ti-6AL-4V-ELI alloy
0.2 % Yield strength ≥ N/mm2 | Tensile strength N/mm2 | Elongation ≥ % | Modulus of elasticity kN/mm2 |
760 | 825-860 | 8-10 | 110 |
Table 4Physical properties of Ti-6AL-4V-ELI alloy
Density g/cm3 | Specific heat capacity J/kg K | Thermal conductivity W/m K | Electrical resistivity Ω mm2/m |
4.45 | 560 | 6.9 | 1.71 |
3. Experimental consideration
Determining the Nanoparticle Volume: Nanoparticle volume was obtained by the initial measurements of its dimension using a Transmission electron microscope (TEM).
If the nanoparticles happen to be spherical, the volume is calculated with Eq. (1) [22]:
where is the sphere radius.
The volume of rod-shaped nanoparticles can be found using Eq. (2):
where for length while for rod radius.
The volume of nanoparticles that are plate-shaped can be found using Eq (3):
where for thickness while for nanoplate radius.
The volume of cube-shaped nanoparticles can be found using Eq. (4):
where is known as cube diameter, software such as MiniTab is used to examine the TEM images in getting the dimensions. Measurement is done through various Transmission electron microscope networks. The average is gotten and put in place appropriately using the above equations. Usually, they can all be gotten using only the transmission electron microscope. The nano-lubricant volume concentration can be calculated using Eq. (5) [23]:
where – mass of nanoparticles, – density of nanoparticles, – mass of lubricant, – density of lubricant. The determine the appropriate quantity of nanoparticles to be mixed with the base oil, Eq. (6) can be used:
where – mass of nanoparticles, and – mass of base oil.
4. Method used for the preparation of nano-lubricant and the machining operation
Ultra-sonication is the chosen method used in this experiment to dispersion the nanoparticles into the base oil. The stages involved in the formulation of the nano-lubricants are as follows:
1) The authors weigh the TiO2 nanoparticle using a digital electronic scale balance. The desired measurement range is 110 mg, and a maximum error of 0.1 mg was permissible.
2) The TiO2 nanoparticles are added to the mineral oil.
3) A homogenized mixture is obtained by oscillating the solution in a Branson ultrasonic bath for 3 hours. Surfactants were not added because the nano lubricants are to be used in the experiments immediately, and adding surfactants may decrease the nano lubricant’s thermal conductivity and performance.
4) There was good stability of the TiO2 nanoparticle in the white mineral oil.
The experiment was carried out with the XM1060 CNC machine, and the experimental setup is shown in Fig. 1.
Fig. 1Schematic diagram of the experimental set-up for machining operations
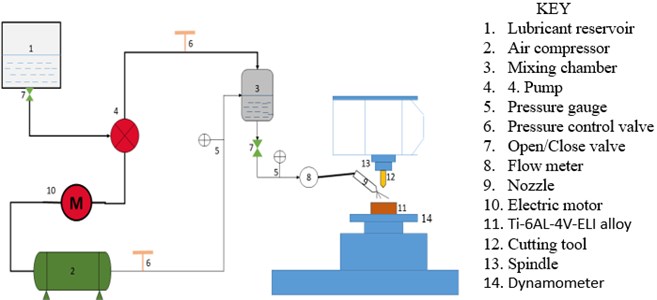
Steps taken for the experimental investigations are as follows:
1) The preparation of the vertical CNC milling machine system ready for performing the machining operation,
2) Fixing the milling cutter of 80 mm diameter with carbide insert on the machine’s spindle taper.
3) The workpiece is mounted, clamped on vice on top of the table of the machine.
4) CNC programs are produced to determine the cutting tool’s travel path. The , , and axes are taken into consideration when programming the machine. Spindle speed, feed rate, axial depth of cut, and radial depth of cut are predetermined and imputed to the machine, then performing end milling operation.
5) A Taguchi orthogonal array is generated in Minitab 16 to give the parameter combination for machining
6) While machining, the cutting forces of the workpiece were measured with the dynamometer.
7) Use the Taguchi method to develop a predictive model for the three (3) machining conditions’ performance in machining operations.
8) The cutting parameters chosen for this experiment are derived from the manufacturer's machining recommendations in Table 5.
Table 5Cutting parameters
Parameter | Units | Level 1 | Level 2 | Level 3 |
Cutting Speed | (rpm) | 2000 | 2500 | 3000 |
Feed rate | (mm/min) | 150 | 200 | 250 |
Depth of cut | (mm) | 0.3 | 0.6 | 0.9 |
Lubrication condition | Dry | Mineral oil | TiO2 Nano-lubricant |
5. Procedure employed to develop the mathematical model
For this experiment, the lubrication conditions (Dry, Mineral oil-lubricant, and Mineral oil-based TiO2 nano-lubricant), Spindle speed, depth of cut, and feed rate are considered the turning parameters. The selection of the machining parameters’ value ranges is selected based on the tool manufacturer’s recommendations. The Taguchi method (L9 orthogonal array) is used to organize the experimental setup for cutting parameters (Spindle speed, feed rate, Depth of Cut, and Lubrication Condition) with three levels (34). Minitab 19 software is used for multiple regression. The response of this study is cutting forces.
Taguchi method is a statistical method with the primary objective of improving quality, and there are two major tools: the orthogonal array and the signal-to-noise ratio. This method significantly reduces the number of experiments, and Taguchi’s method accelerates the experimental work. This process is essential for robust experimental design. The optimal setting is the parameter combination, which has the highest S/N ratio. The Taguchi method is known to discover control factors, i.e., the operating parameters [24]. The S/N ratio represented as can be calculated using Eq. (7):
There are three S/N ratio characteristics in this optimizing process, i.e., the lower, the better; the higher, the better, and the nominal-the better. Nevertheless, in this experiment, the cutting force is best minimized, so the lower, the better ratio is selected. Therefore, in modifying the observed data, the lower-the-better type S/N ratio is represented in Eq. (8) [25]:
where being the S/N ratio for the lower-the-better characteristic, represents the measured quality characteristic for the th repetition and, , the number of repetitions in a trial. The subsequent steps for predicting and checking the originality of quality character development occur when established design parameter levels assign the highest design parameter. The approximate number ratio can be calculated using Eq. (9):
Taguchi Array L9(34), Factors 4, and 9 Runs are applied in this study.
6. Results and discussion
In this section, the Analysis of Signal-to-Noise-Ratio and Analysis of Variance (ANOVA) is applied for the statistical analysis of the experimental results. The Taguchi-based orthogonal experiment was carried out, and the results were recorded and tabulated in Table 6. In Table 7, Cutting Speed, Feed rate, Depth of cut, Lubrication conditions are ranked in order of significance to reducing cutting forces. The Lubrication condition has a delta score of 5.89, followed by the cutting speed of 2.32, feed rate of 2.02, and depth of cut having a delta score of 1.08. Hence, there is an exponential change in the cutting forces when the lubrication conditions are changed. The results show a reduction in cutting forces when cutting speed increases from 2500 to 3000 rpm, the feed rate reduces from 300 to 150, and the depth of cut. Still, when the cutting speed is constant, the feed rate varies from 150 to 200 mm/min, and the depth of cut is changed from 0.9 to 0.3 mm under the nano-lubricants. There is a far more significant reduction in cutting force, based on Figs. 2 and 3, with optimum cutting parameters. As cutting Speed of 3000 rpm, feed rate of 200 mm/min, depth of cut of 0.3 mm, and machining with the Nano-lubricant. Table 8 also shows the response mean analysis.
Table 6Experimental results of cutting force during the machining of Ti-6AL-4V-ELI alloy
Exp. runs | Cutting speed (rpm) | Feed rate (mm/min) | Depth of cut (mm) | Lubrication condition | Cutting force (N) |
1 | 2000 | 150 | 0.3 | 1 | 80 |
2 | 2000 | 200 | 0.6 | 2 | 65 |
3 | 2000 | 300 | 0.9 | 3 | 56 |
4 | 2500 | 150 | 0.6 | 3 | 35 |
5 | 2500 | 200 | 0.9 | 1 | 75 |
6 | 2500 | 300 | 0.3 | 2 | 70 |
7 | 3000 | 150 | 0.9 | 2 | 58 |
8 | 3000 | 200 | 0.3 | 3 | 30 |
9 | 3000 | 300 | 0.6 | 1 | 75 |
Table 7Response for signal to noise ratio (Smaller is better for the operations)
Level | Cutting speed | Feed rate | Depth of cut | Machining condition |
1 | –36.43 | –34.74 | –34.84 | –37.69 |
2 | –35.09 | –34.43 | –34.88 | –36.14 |
3 | –34.10 | –36.46 | –35.91 | –31.80 |
Delta | 2.32 | 2.02 | 1.08 | 5.89 |
Rank | 2 | 3 | 4 | 1 |
Table 8Response for the means: smaller is better during operations
Level | Cutting speed | Feed rate | Depth of cut | Machining condition |
1 | 67.00 | 57.67 | 60.00 | 76.67 |
2 | 60.00 | 56.67 | 58.33 | 64.33 |
3 | 54.33 | 67.00 | 63.00 | 40.33 |
Delta | 12.67 | 10.33 | 4.67 | 36.33 |
Rank | 2 | 3 | 4 | 1 |
ANOVA gives the understanding of the input data and results precisely after a continuing series of trials. Table 9 shows the ANOVA results with the cutting force. % represents the percentage of contribution of each factor on the total variation indicates the degree of influence on the result of the cutting force [26]. According to Table 9, the Cutting Speed, Feed rate, Depth of Cut, and Machining conditions have contribution percentages of 9.55 %, 6.44 %, 0.54 %, and 78.63 %. The model developed predicted the cutting force with 95.17 % having a 4.82 % error, which shows the high accuracy of the machining experimental results alongside the predicted results. The Machining condition is shown to have the highest contribution to the cutting force.
Table 9ANOVA for the cutting force
Source | DF | Adj S.S. | Adj MS | % Contribution | F-Value | P-Value |
Regression | 4 | 2396.63 | 599.16 | 95.17 | 19.71 | 0.007 |
Cutting speed (rpm) | 1 | 240.67 | 240.67 | 9.55 | 7.92 | 0.048 |
Feed rate (mm/min) | 1 | 162.29 | 162.29 | 6.44 | 5.34 | 0.082 |
Machining condition | 1 | 1980.17 | 1980.17 | 78.63 | 65.14 | 0.001 |
Depth of cut (mm) | 1 | 13.5 | 13.5 | 0.54 | 0.44 | 0.542 |
Error | 4 | 121.6 | 30.4 | 4.82 | ||
Total | 8 | 2518.22 |
Table 10The summary of the cutting force model
S | R-sq | R-sq(adj) | R-sq(pred) |
5.51351 | 95.17 % | 90.34 % | 76.01 % |
Table 11Coefficients of the cutting force study
Term | Coef. | S.E. Coef. | T-Value | P-Value | VIF |
Constant | 110.7 | 14.5 | 7.61 | 0.002 | |
Cutting speed (rpm) | –0.01267 | 0.00450 | –2.81 | 0.048 | 1.00 |
Feed rate (mm/min) | 0.0681 | 0.0295 | 2.31 | 0.082 | 1.00 |
Machining condition | –18.17 | 2.25 | –8.07 | 0.001 | 1.00 |
Depth of cut (mm) | 5.00 | 7.50 | 0.67 | 0.542 | 1.00 |
Fig. 2Residual plot for cutting force showing normal probability plot, fits, frequency, and observation order
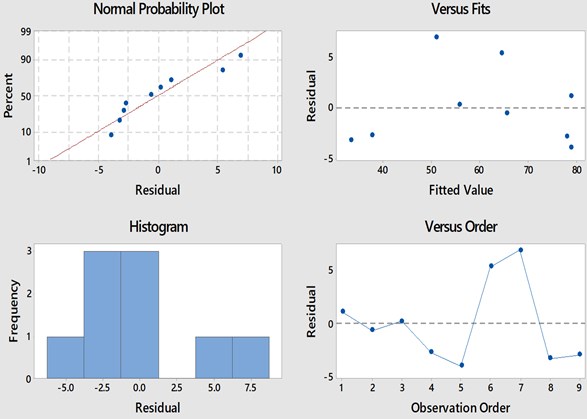
The Regression model is a mathematical equation used to estimate relationships between a dependent variable and one or more independent variables [27-29]. In the regression model for the cutting force Eq. (10), the dependent variable is the cutting force. The independent variables are Cutting Speed, Feed rate, Depth of cut, and machining conditions. Table 11 shows the coefficients relationship of the machining parameters and the cutting force. The variance inflation factor (VIF) proves that the relationship is normal with a multicollinearity set of 1. The P-value from Table 9 shows that the cutting speed is significant with a value of 0.048, and the most significant parameter is the machining condition with 0.001:
Cutting Force (N) = 110.7 – 0.01267 Cutting Speed (rpm) + 0.0681 Feed Rate (mm/min) – 18.17 Machining Condition + 5.00 Depth of Cut (mm).
Fig. 2 shows the residual plot for Cutting force; this is a four in one graph derived with the help of the Minitab 16 software. The normal probability plot predicts the cutting force with the cutting parameters. The histogram shows the frequency of occurrence, and the versus order shows the residual against the observation runs. Based on the analysis, it can be said that the Taguchi design of the experiment is linear.
7. The effects of cutting speed, feed rate, and depth-of-cut and their interaction on the cutting force under the three cutting conditions
The line plot represents the individual variable’s influence on the cutting force, resulting in three (3) cutting conditions. The Line plot of the effects of cutting speed, feed rate, and depth of cut on the cutting force is represented in Figs. 3-5. The result analysis in Fig. 3 shows the effect of cutting speed on the cutting force under the three different lubricating conditions. Furthermore, the graph showed that the minimum cutting force was obtained at the highest cutting speed for all three cutting conditions.
Fig. 3Effect of cutting speed (rpm) on cutting force (N) under the three machining conditions
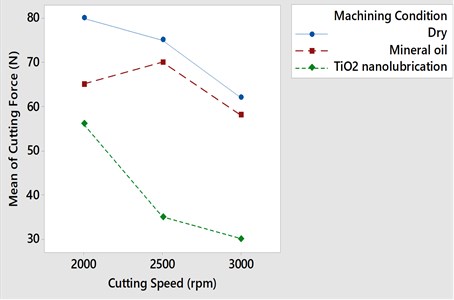
This result has proven that cutting speed has little or no effect in increasing cutting force during the cutting process via milling machining. Moreover, the nano-lubricant machining condition has a minimum cutting force of 30 (N). Fig. 4 demonstrates the effect of depth of cut on the cutting force under the three different lubricating conditions. The plot shows that the lowest cutting force when the feed rate is 200 (mm/min). In Fig. 5, it is shown that there was a sudden increase of the cutting force at the TiO2 nano-lubrication condition. This can result from vibration coursed by chips falling back to the cutting region [30]. However, it still has the lowest cutting force obtained when the depth of cut is at 0.3mm, and the nano lubricant is applied. However, from the literature, depth of cut is not a friendly parameter to cutting force. Therefore, applying the depth of cut in cutting Ti-6AL-4V-ELI alloy needs a critical study. It is preferable to apply nano-lubricant with excellent chemical and mechanical properties.
The interaction analysis is another way of studying the relationship between two factors concerning the response value. For example, Fig. 6 illustrates all the cutting parameter’s interactions on the cutting force. The cutting force is increased when the machining condition has not interacted with the feed rate. The Interaction Plot is designed to show the relationship between individual factors concerning the cutting force. From Fig. 6, when the cutting speed is at 3000 rpm and the Feed rate is 200 mm/min, the cutting force reduces to its lowest. This proves that high cutting speed with low feed rate interactions leads to minimum cutting force during machining of Ti-6AL-4V-ELI alloy. Because the application of the nanoparticles in the mineral oil increases the mechanical property of the base oil, which significantly improves the machining condition during operation to reduce vibration and friction during the face-milling machining, this result is in line with [25, 31-33]. The vibration occurs due to the unwanted movement of the chips being removed from the workpiece in the machining process and can affect the workpiece during the machining operation. Therefore, the TiO2 nano-lubricant in this study assists in removing the chips from the machining region, thereby reducing the cutting force. This reduction process of the cutting force also will assist in prolonging the cutting tool life during operation [34-36].
Fig. 4Effect of feed rate (mm/min) on cutting force ((N) under the three lubrication conditions
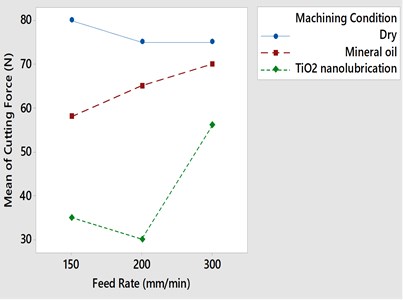
Fig. 5Effect of depth of cut (mm) on cutting force ((N) under the three lubrication conditions
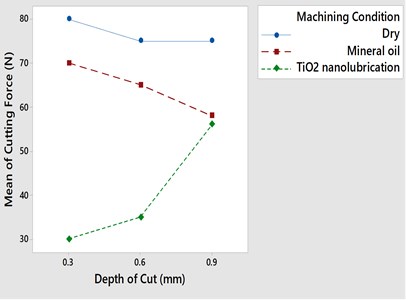
Fig. 6Interaction plot for the cutting force under the three machining conditions with the cutting parameters
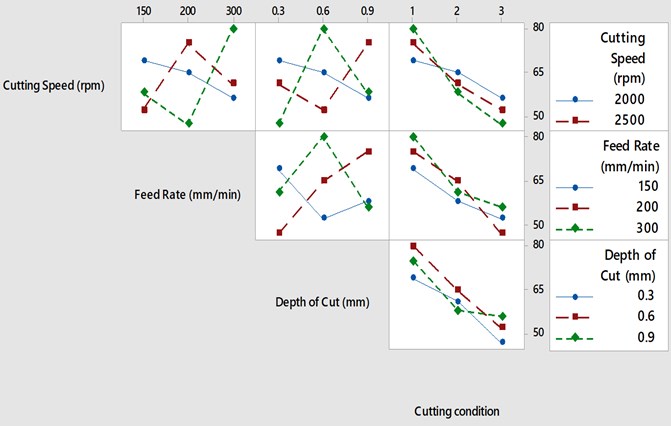
8. Conclusions
In this research, the effect of the dry, mineral oil, and TiO2 nano-lubricants on the cutting forces in the machining of Grade 23 Titanium alloy (Ti-6Al-4V) was investigated. The experiment was carried out using the Taguchi experimental design and dynamometer to measure the cutting force during the machining process, and the experimental results were analyzed using Minitab 16. The research considered four parameters such as cutting speed, feed rate, depth-of-cut, and lubrication cutting conditions on the response (cutting force).
This study has the following conclusions:
1) Mineral oil-base-TiO2 nano-lubricant was responsible for the reduction of the cutting force by 19 % compared to mineral oil and the dry machining operations
2) The cutting speed increase with a relatively low feed rate leads to cutting force reduction
3) The optimal parameters with the minimum cutting force when milling Ti-6AL-4V-ELI alloy are cutting speed at 3000 rpm, 200 mm/min feed rate, 0.3 mm depth of cut, under the machining with Mineral oil-base-TiO2 nano-lubricant with a minimum cutting force of 30 N.
4) The most significant parameters are the machining condition with a 0.001 P-value, with a high contribution ratio of 78.63 %. However, the least significant parameter is the depth of cut with a P-value of 0.542 and a contribution ratio of 0.54 %.
References
-
A. Rizzo et al., “The critical raw materials in cutting tools for machining applications: a review,” Materials, Vol. 13, No. 6, p. 1377, Mar. 2020, https://doi.org/10.3390/ma13061377
-
D. Y. Pimenov et al., “Improvement of machinability of Ti and its alloys using cooling-lubrication techniques: a review and future prospect,” Journal of Materials Research and Technology, Vol. 11, pp. 719–753, Mar. 2021, https://doi.org/10.1016/j.jmrt.2021.01.031
-
H. Hegab, U. Umer, I. Deiab, and H. Kishawy, “Performance evaluation of Ti-6Al-4V machining using nano-cutting fluids under minimum quantity lubrication,” The International Journal of Advanced Manufacturing Technology, Vol. 95, No. 9-12, pp. 4229–4241, Apr. 2018, https://doi.org/10.1007/s00170-017-1527-z
-
H. Attar, S. Ehtemam-Haghighi, N. Soro, D. Kent, and M. S. Dargusch, “Additive manufacturing of low-cost porous titanium-based composites for biomedical applications: Advantages, challenges and opinion for future development,” Journal of Alloys and Compounds, Vol. 827, p. 154263, Jun. 2020, https://doi.org/10.1016/j.jallcom.2020.154263
-
A. M. Khan et al., “Energy-based cost integrated modelling and sustainability assessment of Al-GnP hybrid nanofluid assisted turning of AISI52100 steel,” Journal of Cleaner Production, Vol. 257, p. 120502, Jun. 2020, https://doi.org/10.1016/j.jclepro.2020.120502
-
I. P. Okokpujie, C. A. Bolu, and O. S. Ohunakin, “Comparative performance evaluation of TiO2, and MWCNTs nano-lubricant effects on surface roughness of AA8112 alloy during end-milling machining for sustainable manufacturing process,” The International Journal of Advanced Manufacturing Technology, Vol. 108, No. 5-6, pp. 1473–1497, May 2020, https://doi.org/10.1007/s00170-020-05397-5
-
G. Gaurav, A. Sharma, G. S. Dangayach, and M. L. Meena, “Assessment of jojoba as a pure and nano-fluid base oil in minimum quantity lubrication (MQL) hard-turning of Ti-6Al-4V: A step towards sustainable machining,” Journal of Cleaner Production, Vol. 272, p. 122553, Nov. 2020, https://doi.org/10.1016/j.jclepro.2020.122553
-
A. N. Mohd Khalil, A. I. Azmi, M. N. Murad, and M. A. Mahboob Ali, “The effect of cutting parameters on cutting force and tool wear in machining nickel titanium shape memory alloy ASTM F2063 under minimum quantity Nanolubricant,” Procedia CIRP, Vol. 77, pp. 227–230, 2018, https://doi.org/10.1016/j.procir.2018.09.002
-
H. Hegab, B. Darras, and H. A. Kishawy, “Sustainability assessment of machining with nano-cutting fluids,” Procedia Manufacturing, Vol. 26, pp. 245–254, 2018, https://doi.org/10.1016/j.promfg.2018.07.033
-
A. A. D. Sarhan, M. Sayuti, and M. Hamdi, “Reduction of power and lubricant oil consumption in milling process using a new SiO2 nanolubrication system,” The International Journal of Advanced Manufacturing Technology, Vol. 63, No. 5-8, pp. 505–512, Nov. 2012, https://doi.org/10.1007/s00170-012-3940-7
-
H. Ghaednia and R. L. Jackson, “The role of nanoparticles in lubricants; performing lubricated and dry friction tests,” Tribology and Lubrication Technology, Vol. 71, No. 7, pp. 20–24, 2015.
-
B. Zareh-Desari and B. Davoodi, “Assessing the lubrication performance of vegetable oil-based nano-lubricants for environmentally conscious metal forming processes,” Journal of Cleaner Production, Vol. 135, pp. 1198–1209, Nov. 2016, https://doi.org/10.1016/j.jclepro.2016.07.040
-
S. Roy, R. Kumar, A. Kumar Sahoo, and R. Kumar Das, “A brief review on effects of conventional and nano particle based machining fluid on machining performance of minimum quantity lubrication machining,” Materials Today: Proceedings, Vol. 18, pp. 5421–5431, 2019, https://doi.org/10.1016/j.matpr.2019.07.571
-
I. P. Okokpujie, C. A. Bolu, O. S. Ohunakin, E. T. Akinlabi, and D. S. Adelekan, “A review of recent application of machining techniques, based on the phenomena of CNC machining operations,” Procedia Manufacturing, Vol. 35, pp. 1054–1060, 2019, https://doi.org/10.1016/j.promfg.2019.06.056
-
S. S. Rahman, M. Z. I. Ashraf, A. N. Amin, M. S. Bashar, M. F. K. Ashik, and M. Kamruzzaman, “Tuning nanofluids for improved lubrication performance in turning biomedical grade titanium alloy,” Journal of Cleaner Production, Vol. 206, pp. 180–196, Jan. 2019, https://doi.org/10.1016/j.jclepro.2018.09.150
-
R. Anand, A. Raina, M. Irfan Ul Haq, M. J. Mir, O. Gulzar, and M. F. Wani, “Synergism of TiO2 and graphene as nano-additives in bio-based cutting fluid-an experimental investigation,” Tribology Transactions, Vol. 64, No. 2, pp. 350–366, Mar. 2021, https://doi.org/10.1080/10402004.2020.1842953
-
G. Li, S. Yi, N. Li, W. Pan, C. Wen, and S. Ding, “Quantitative analysis of cooling and lubricating effects of graphene oxide nanofluids in machining titanium alloy Ti6Al4V,” Journal of Materials Processing Technology, Vol. 271, pp. 584–598, Sep. 2019, https://doi.org/10.1016/j.jmatprotec.2019.04.035
-
M. Shnfir, O. A. Olufayo, W. Jomaa, and V. Songmene, “Machinability study of hardened 1045 steel when milling with ceramic cutting inserts,” Materials, Vol. 12, No. 23, p. 3974, Nov. 2019, https://doi.org/10.3390/ma12233974
-
A. Anand, K. Vohra, M. I. Ul Haq, A. Raina, and M. F. Wani, “Tribological considerations of cutting fluids in machining environment: a review,” Tribology in Industry, Vol. 38, No. 4, pp. 463–474, Dec. 2016.
-
I. P. Okokpujie et al., “Experimental and mathematical modeling for prediction of tool wear on the machining of aluminium 6061 alloy by high speed steel tools,” Open Engineering, Vol. 7, No. 1, pp. 461–469, Dec. 2017, https://doi.org/10.1515/eng-2017-0053
-
Y. Chen, P. Renner, and H. Liang, “Dispersion of nanoparticles in lubricating oil: a critical review,” Lubricants, Vol. 7, No. 1, Jan. 2019, https://doi.org/10.3390/lubricants7010007
-
P. Yang, Q. Zeng, K. Dong, and H. Zhu, “A quick method for developing interparticle force models of spherical gold nanoparticles from molecular dynamics simulation,” Powder Technology, Vol. 362, pp. 501–506, Feb. 2020, https://doi.org/10.1016/j.powtec.2019.10.105
-
I. P. Okokpujie, O. S. Ohunakin, and C. A. Bolu, “Multi-objective optimization of machining factors on surface roughness, material removal rate and cutting force on end-milling using MWCNTs nano-lubricant,” Progress in Additive Manufacturing, Vol. 6, No. 1, pp. 155–178, Feb. 2021, https://doi.org/10.1007/s40964-020-00161-3
-
S. M. Ravi Kumar and S. K. Kulkarni, “Analysis of hard machining of titanium alloy by Taguchi method,” Materials Today: Proceedings, Vol. 4, No. 10, pp. 10729–10738, 2017, https://doi.org/10.1016/j.matpr.2017.08.020
-
Imhade P. Okokpujie, Salawu Enesi, Obinna N. Nwoke, Ugochukwu Okonkwo, and Kennedy Okokpujie, “Effects of process parameters on vibration frequency in turning operations of perspex material,” Lecture Notes in Engineering and Computer Science, Vol. 2236, pp. 700–707, Jul. 2018.
-
M. Li, T. Yu, L. Yang, H. Li, R. Zhang, and W. Wang, “Parameter optimization during minimum quantity lubrication milling of TC4 alloy with graphene-dispersed vegetable-oil-based cutting fluid,” Journal of Cleaner Production, Vol. 209, pp. 1508–1522, Feb. 2019, https://doi.org/10.1016/j.jclepro.2018.11.147
-
I. P. Okokpujie, O. O. Ajayi, S. A. Afolalu, A. A. Abioye, E. Y. Salawu, and M. Udo, “Modeling and optimization of surface roughness in end milling of aluminium using least square approximation method and response surface methodology,” International Journal of Mechanical Engineering and Technology (IJMET), Vol. 9, No. 1, pp. 587–600, 2018.
-
Q. Chen, B. Xue, and M. Zhang, “Improving symbolic regression based on correlation between residuals and variables,” in GECCO’20: Genetic and Evolutionary Computation Conference, pp. 922–930, Jun. 2020, https://doi.org/10.1145/3377930.3390161
-
O. I. P., “Experimental analysis of the influence of depth of cut, time of cut, and machining speed on vibration frequency during turning of Al1060 alloy,” International Journal of Advanced Trends in Computer Science and Engineering, Vol. 9, No. 4, pp. 6783–6789, Aug. 2020, https://doi.org/10.30534/ijatcse/2020/377942020
-
R. Naveen Anthuvan, S. Pratheesh Kumar, R. Arun Prakash, B. Arunkarthik, and A. Akhilesh, “Machinability study in milling of Ti-6Al-4V using cryogenic treated and coated tool,” Materials Today: Proceedings, Vol. 46, pp. 8417–8428, 2021, https://doi.org/10.1016/j.matpr.2021.03.451
-
A. M. Khan et al., “Energy, environmental, economic, and technological analysis of Al-GnP nanofluid – and cryogenic LN2-assisted sustainable machining of Ti-6Al-4V alloy,” Metals, Vol. 11, No. 1, p. 88, Jan. 2021, https://doi.org/10.3390/met11010088
-
I. P. Okokpujie, O. S. Ohunakin, C. A. Bolu, and K. O. Okokpujie, “Experimental data-set for prediction of tool wear during turning of al-1061 alloy by high speed steel cutting tools,” Data in Brief, Vol. 18, pp. 1196–1203, Jun. 2018, https://doi.org/10.1016/j.dib.2018.04.003
-
M. H. Sulaiman, N. A. Raof, and A. N. Dahnel., “The investigation of PVD coating, cryogenic lubrication and ultrasonic vibration on tool wear and surface integrity in manufacturing processes.,” Journal Tribology, Vol. 28, pp. 105–116, 2021.
-
R. Anand, M. I. Haq, and A. Raina, “Bio-based nano-lubricants for sustainable manufacturing,” Nanomaterials and Environmental Biotechnology, Vol. 22, pp. 333–380, 2020.
-
A. Raina, M. Irfan Ul Haq, A. Anand, and J. Sudhanraj, “Lubrication characteristics of oils containing nanoadditives: influencing parameters, market scenario and advancements,” Journal of The Institution of Engineers (India): Series D, Vol. 102, No. 2, pp. 575–587, Dec. 2021, https://doi.org/10.1007/s40033-021-00272-3
-
R. Anand, A. Raina, M. I. Ul Haq, M. Fadzli, and B. Abdollah, “Minimum quantity lubrication for sustainable manufacturing,” in Tribology and Sustainability, Boca Raton: CRC Press, 2021, pp. 269–292, https://doi.org/10.1201/9781003092162-18
Cited by
About this article
The authors wish to recognize the University of Johannesburg, Johannesburg, 2028, South Africa Management, to fund the publication fee.