Abstract
Using frequency splitting, two energy management strategies (EMS) based on Haar wavelet decomposition and Fourier analysis for fuel cell hybrid vehicle (FCHV) are proposed to manage efficiently the power flow between components. The paper aims to discuss the performances of the proposed EMS in terms of dynamic behavior, robustness operation, real time application and fuel economy. For apply this methodology, two EMS approaches are elaborated and successfully tested for parallel Fuel Cell/UC: conventional approach using Fourier Transform analysis (FT) and Wavelet analysis approach allowing natural frequency splitting. Finally, and to evaluate the performance and relevance of the developed approach, a comparison analysis were conducted. The simulation results exhibit the effectiveness of both strategies. Indeed, Wavelet analysis leads to better results in terms of energy flow and dynamic behavior, excellent robustness and stability of system, as well as energy economy improvement. A very relevant strategy is proposed based on Wavelet analysis using digital filtering techniques, which enables a natural frequency splitting to ensure the best global performances. In addition, the approach remains simple and suitable for real time operation.
1. Introduction
Fuel Cell Hybrid Vehicles (FCHV) have environmental and health benefits compared to conventional vehicles. They also have the capacity to make a real solution to the limits of existing cleaner ones: hybrid electric vehicles (HEV), plug-in hybrid electric vehicles (PHEV), electric vehicles with range extender and battery electric vehicles (BEV). However, their main impact is extremely dependent how to choose at each moment the best supply of power between the different energy sources [1, 2]. The combination of the proton exchange membrane (PEM) fuel cell (PEM) with ultra-capacitor (UC) is one of the most promising technologies for FCHV, therefore gaining attraction since it makes it possible to enhance his capability to upraise power and energy densities and moreover becomes possible to recover the total of the braking energy [3, 4].
EMS grows to be one of the best issues for traction application using hybrid power source. It assures management of the power division between various energy sources and the load requirement therefore gaining efficiency and performance of our system [3-5].
In literature, EMS has been considered in several works [5-23]; we can classify these strategies into two main categories:
First: strategies based on predefined rules, main objective of this method is to operate the system at its point of return with advantage of being more simply implementable in real time, and these strategies do not require a priori knowing of driving cycle in advance. There are two types of rules: deterministic rules and fuzzy logic-based rules as examples for deterministic rules such as: thermostat, power follower, frequency-based, engine optimal working point, engine optimal operation line, engine optimal efficiency region and system optimal operation point [5-12], the results of those strategies show that they have better fuel economy and vehicle mileage.
A non-linear approach such as Passivity Based Control PBC methodology enables to guarantee the asymptotic stability of the control/system combination, another interesting approaches of energy management in hybrid electric vehicles are artificial intelligence (fuzzy and neural network), it has the necessary robustness to naturally take into account system variations, but they also require accurate sizing of the power sources with a risk of over sizing. This leads to additional costs (real and computational) and decreases system reliability which limits their real time functioning and industrial integration [13-17].
Second: strategies based on optimization approaches was another method can say more important because a series of optimization techniques have been applied in recent years such as linear programming or non-linear programming, dynamic programming, optimal linear-quadratic control, the genetic algorithm and Model Predictive Control (MPC) [18-20], moreover Pontriagin minimum principle has been used to achieve online optimum power distribution in a hybrid vehicle, Another online energy management strategy called Equivalent Consumption Minimization Strategy “ECMS”, proposed by Musardo and Serrao [21-23], is based on an adaptive algorithm, In addition, these methods being global, it is very difficult to extract information from them about optimization methods that can be generalized to different situations or configurations. Their performances are related to the tradeoff between complexity formulation (parameters/constraints/objectives), conflicting requirements, computational time and exploration abilities (poor convergence). In addition, necessity to know the trip information of driving cycle a priori on the basis of expertise or on the analysis of the behavior of system components which restricts their real time integration.
Lastly, a suitable EMS based on frequency decomposition approach [24, 25] that present excellent performances by sharing power and energy requirements. Conventional approaches uses bandwidth filter with Fourier analysis to make a frequency splitting of the power requirement. They lead to introduce a delay in system reaction increasing the risk to damage the system and decrease his reliability. Thus, for this reason, EMS based on wavelet approach allowing natural frequency splitting is well adapted to this strategy specification [26, 27].
Wavelet approach came into effect before than 30 years ago in many domains mostly reserved for the mathematics field but the correlation with filtering behavior allows adopting wavelet in signal processing. This concept allowing exploring the signal and its characteristics at different scales which seems to be suited for power split in hybrid energy sources. Wavelet-based EMS becomes very successfully technique for this domain [28-30], gratefulness to S. Mallat [31] who developed the concept of multi-resolution representation and capacity in analysis and acquisition of rapid and transient frequency during the drive cycle, without slop limitation and response delay.
Surroundings this research, to find the most suitable energy management technique for an FC/UC hybrid vehicular power system, an effective and robust method based on wavelet approach is proposed, (exactly discrete wavelet transform) and also compared with classical Fourier analysis.
Simulation results are presented to compare wavelet approach with Fourier analysis. Our interest is focused on energy flow and dynamic behavior, robustness and stability of system, as well as energy economy improvement.
By using two different driving cycles, results provide evidence the strength of this approach and show high performances of the elaborated control method for various load power demands (driving cycles).
2. Energy management strategy
2.1. Description of the strategy
Hybrid power system is designed based on the power and energy requirement, their EMS leads mainly to maximize fuel economy, minimize emissions, minimize system cost and achieve good driving performance
For this purpose, and to coordinate between the energy sources and offer the power flow control for the mechanical and electrical path, an appropriate EMS is proposed to consider the specific characteristics of system components (fuel cell () dynamics, ultra-capacitor () state of charge (), efficiency improvement, transient performance, recovering mode …).
Wavelet frequency decomposition is highly perfect method for frequency splitting for achieve to perfect EMS, its main principle is based on the use of the (the fastest energy source) for supplying the high band of the load power frequency spectrum (), thus, avoiding the fuel starvation problem and permitting to propose a downsized design. Conversely, low frequencies () are provided by the fuel cell, which contributes to the long-term autonomy and also maintain the state of charge ().
The configuration of hybrid vehicle topology need to control unit of the vehicle for that we use an efficient adaptable control scheme based on a cascaded control loop, as depicted in Fig. 1.
Our hybrid vehicle system is composed on two different sources fuel cell () and ultra-capacitors (), each source is connected by a converter, for this we have exploited a strong strategy based on wavelet transform to ensure the sharing of power demand and energy between these sources, therefore identify the current trajectory for each source ( and ).
This strategy calls for local control of converters current with closed loop for our system. Thus, to monitor the current trajectory of each source, we have designed a classic proportional integrator controller for each closed current loop.
Fig. 1General chart view of proposed energy management strategy
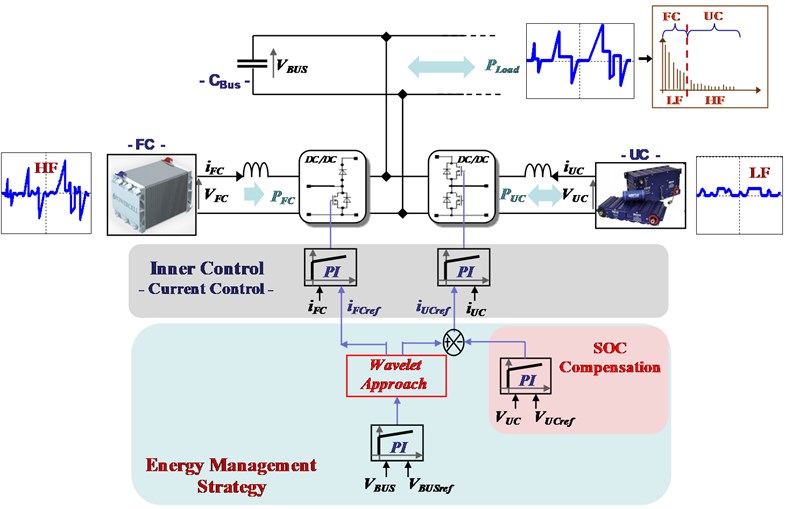
This control strategy estimates future power demand using the bus voltage fluctuations, hence a second Proportional Integrator () controller was exploited for allows to generate the load current estimated which represents the power demand trajectory since is constant (Fig. 1).
Once the power demand is estimated, the proposed EMS remains to be integrated using wavelet approach.
2.2. Driving cycles
Driving cycle generally represents a set of vehicle speed points as a function of time. It is used to assess a vehicle’s fuel consumption and pollutant emissions in a standardized way, so that different vehicles can be compared with each other.
Many driving cycles are regulated in the European Union, in the United States and in Japan and are called standard driving cycles.
In Europe, the most used are UDC (urban drive cycle) or called ECE-15, EUDC (Extra-Urban Driving Cycle), and NEDC (New European Driving Cycle). The last one is the official cycle to standardize pollutant emissions and vehicle autonomy in Europe; it is made up of an urban part called (ECE), which is repeated four times, and an extra-urban part (EUDC).
In our paper, two driving cycles was tested for comparison: UDC and real driving cycle based on NEDC with taking into account a real world driving schedule, experimentally measured, the following figures show the power load profile of each cycle.
Fig. 2Power profile for UDC driving cycle
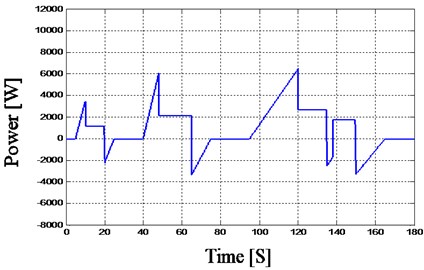
Fig. 3Power profile for REAL driving cycle
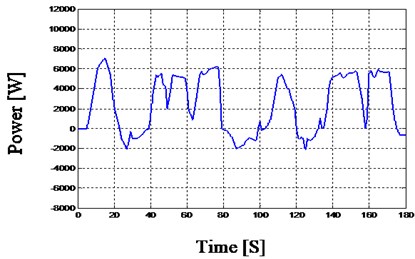
2.3. Wavelet approach
Nowadays time-frequency representations becomes necessary approach in condition monitoring and signal processing to afford both time and frequency information of the analysis signals, most related approach is wavelet transform as powerful approach for extracting features from the transient signal.
Whenever we want talk about wavelet transform, we have to do small degression toward short-time Fourier transform (STFT).
STFT introduced by Denis Gabor in 1946 and as usually sine and cosine functions are used as base signals for the analysis, their time-span from to making them undesirable for transient analysis and also with a fixed window size, in opposite wavelet transform uses a large library of wavelet functions characterized mainly by limited time span and various wave shapes that are appropriate for transient analysis and moreover with a variable size window length allows to give finest time and frequency resolution compared to STFT [28-31].
Consequently, the wavelets transform count as powerful approach in signal processing, in our work we use discrete wavelet transform for frequency decompositions of original signal into two signals one is called signal Approximation with low frequencies and second is called signal detail with high frequencies, the next paragraph reveals theoretical detail about discrete wavelet transform DWT.
2.4. Discrete wavelet transform
Discrete wavelet transform (DWT) use a scale factor and a discretized translation. We call dyadic discrete wavelet transform any wavelet basis working with a scale factor .
Discrete wavelet transform decomposes the sampled input signal, into Approximation signals at a specific level, and Detail signals, as show in Fig. 4.
Fig. 4Standard discrete wavelet decomposition
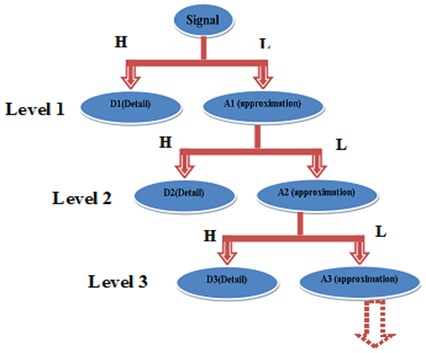
The signal is decomposed as follow:
1) Approximation signal gotten by passing the original signal into high pass filters to analyze the high frequencies ().
2) Detail signal gotten by passing the original signal through a series of low pass filters to analyze the low frequencies ().
The Eq. (1) and Eq. (2) express that decomposition:
where is the approximation of the level immediately above level , where is the length of the filter vector [28-30].
Three steps are necessary in our wavelet decomposition for reach to efficient management strategy:
1) First is Mother wavelet choice for decomposition is Haar, Haar characterized by great characteristic that is the only symmetric wavelet with a compact support can easily decomposes signals into low-pass and high pass components sub-sampled by 2
2) Second: number of level decomposition is three levels using Haar wavelet transform [31].
3) Third is final signal was obtained through reconstruction based on summation of decomposition signals (details and approximation), the particular characteristic of Haar basis is capable to synthesized with perfect reconstruction filters having a linear phase.
Using Haar wavelet can appropriately reach to a perfect EMS, with easy structure and architecture; however, attain our main aim of control.
3. Simulation results
With the aim of testing the energy management strategy proposed in this paper, we divide our simulation results into two parts: first part concern urban driving cycle UDC show in Fig. 5-9, then second part concern Real driving cycle show in Fig. 10-14, the main idea here is how to provide our EMS efficiency based on wavelet approach comparing with classical Fourier approach, the results was carried out on Matlab Simulink and Sim Power Systems Toolboxes, the following data presented in Table 1 represent the electrical characteristic of hybrid system used in this simulation.
Table 1Electrical characteristic of FC-UC hybrid system
Fuel cell | Value |
Open circuit voltage | 150 V |
Rated voltage | 90 V |
Rated current | 90A |
Ultracapacitor | Value |
Capacitance | 47 F |
Rated voltage | 180 V |
Rated current | 120 A |
Optimal voltage () | 150 V 0.26 Ohm |
Inductors and capacities | Value |
Inductors | 300/450 μH |
Rated currents | 150/200 A |
Capacities | 14 mF |
Optimal DC-bus voltage () | 410 V |
Before starting interpretation of our results we must specify the key variable responses of our hybrid system which can show this difference: Load power (), Fuel cell power () and ultra-capacitors power (), currents (, ), UC currents (, ), bus voltages (, ) and voltages (, ).
3.1. Result under UDC driving cycle (wavelet Vs FFT)
All figures of last variables already mentioned are depicted in Fig. 5-9, for UDC driving cycle. and Fig. 10-14 for Real driving cycle, it is important to say that the value of , voltage reference is 150 V and bus voltage reference is 410 V.
Fig. 5Hybrid system response under UDC driving cycle (wavelet vs FFT)
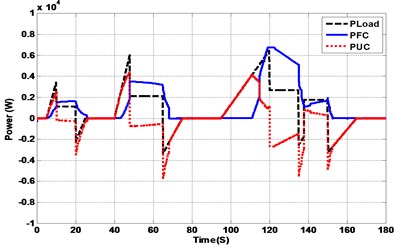
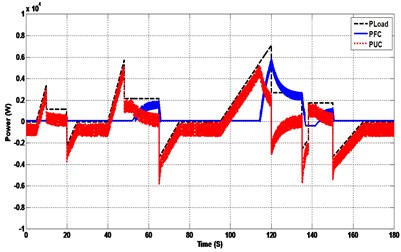
Fig. 6Hybrid system response under UDC driving cycle (wavelet vs FFT)
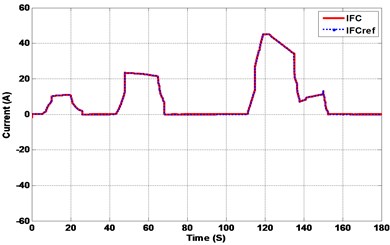
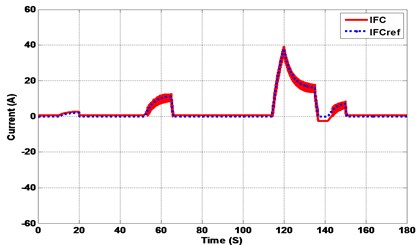
Fig. 7Hybrid system response under UDC driving cycle (wavelet vs FFT)
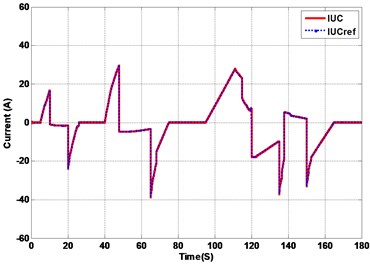
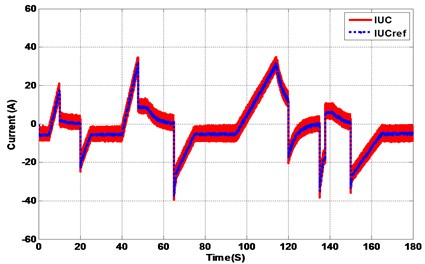
Fig. 8Hybrid system response under UDC driving cycle (wavelet vs FFT)
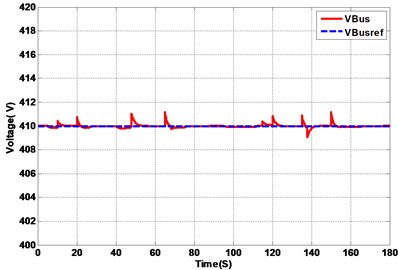
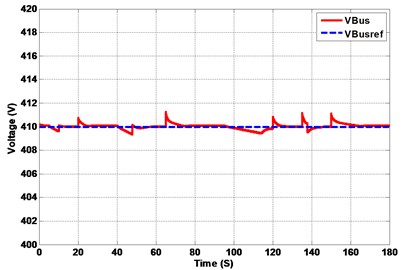
Fig. 9Hybrid system response under UDC driving cycle (wavelet vs FFT)
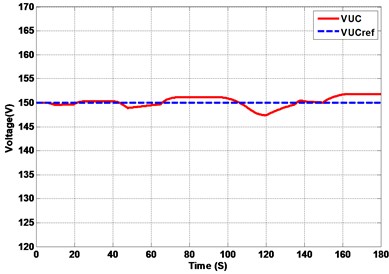
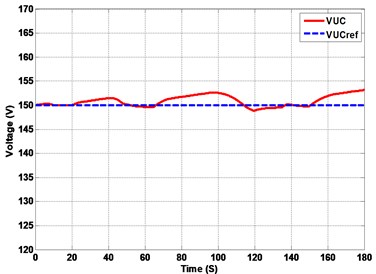
3.2. Result under real driving cycle (wavelet vs FFT)
Figs. 5-14 shows clearly the difference through its waveform responses between two EMSs based one on wavelet approach (left side) and second based on Fourier approach (right side).
Fig. 10Hybrid system response under real driving cycle (wavelet vs FFT)
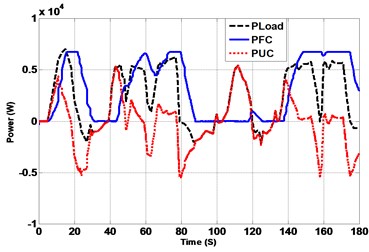
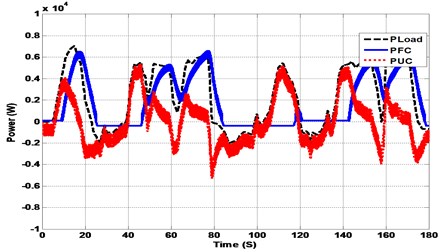
By comparing wavelet analysis (left side) vs Fourier analysis (right side), power responses show (Fig. 5 and Fig. 10) that the EMS imposes to each source respecting its own characteristics.
Fig. 11Hybrid system response under real driving cycle (wavelet vs FFT)
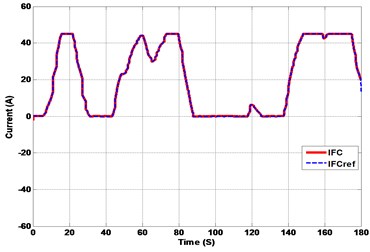
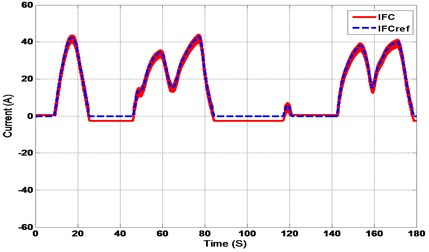
Back to Fig. 1 and in order to protect the converters as well as the sources (UCs and FC) against over currents, the installation of internal current loops which allow the current of each source to be conducted is a necessity
FFT analysis of current , exhibits more oscillations compare to as given away in right side of Fig. 6 and Fig. 11.
Although in our study, we opted for the Proportional-Integral () control structure to ensure robustness to load variations, we can spot proper the wavelet method implemented for frequency splitting has perfect advantages over simulation results:
First: for current behaviour () as a low band pass filter shown in left side of Fig. 6 and Fig. 11.
Second: for current behaviour () as a high-band pass filter shown in left side of Fig. 7 and Fig. 12.
Fig. 12Hybrid system response under real driving cycle (wavelet vs FFT)
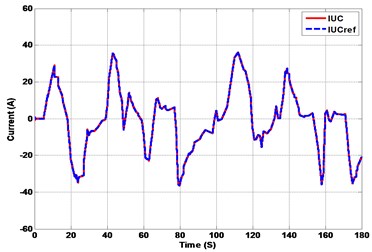
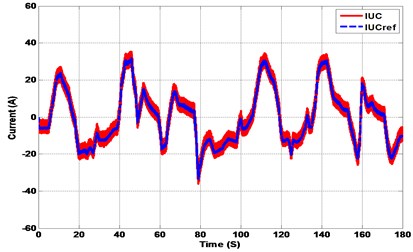
Fig. 13Hybrid system response under real driving cycle (wavelet vs FFT)
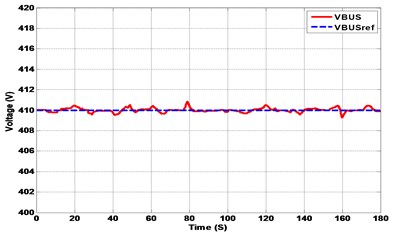
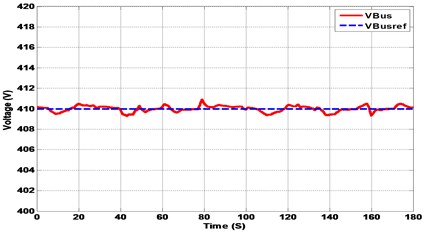
Fig. 14Hybrid system response under real driving cycle (wavelet vs FFT)
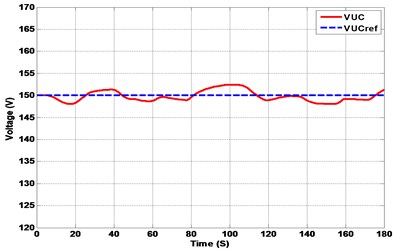
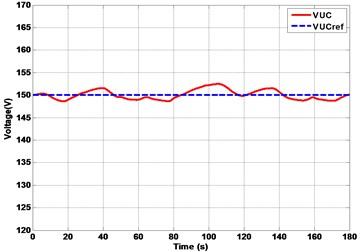
As shown in (Fig. 8 and Fig. 13), power load affect the link voltage transiently with a slight deviation because of wavelet approach characteristic, overshoot less than 1 %, the reference 410 V is followed perfectly).
In opposite side and in both driving cycle using FFT analysis, follows 410 V with small steady state error.
Ultra-capacitors can react quickly to sudden transients in the current of the load; these transients are induced by bus voltage regulation and allow insurance of the major part of the transient component of the demanded power. Energy transfer from to the bus therefore operates correctly and compensates for the energy that is not supplied by the Fuel cell. This allows the battery to react without abrupt variations in its current to the load requests.
Then, as the current of the increases, the discharge of the characterized by the decrease in its tension attenuates until it is cancelled. A rebalancing regime is then established (called compensation), characterized here by recharging the bank of to its reference value () fixed at 150 V (Fig. 9 and Fig. 14). In addition, the system control reacts perfectly by following the trajectory currents (Fig. 6 and Fig. 11) and (Fig. 7 and Fig. 12).
In addition, Wavelet analysis provides hydrogen consumption saving (13 % under UDC driving cycle and 16 % under real driving cycle) which makes it relevant regarding fuel economy.
4. Conclusions
The clarification of the efficiency of wavelet technique in frequency splitting and compare it with Fourier analysis for EMS is role of two on one that is an efficient energy management strategy, by this comparison we illustrate why and witch type of wavelet we used for reach our aim of EMS.
The choice of our technique is not enough without strong, simple architecture and robust energy management strategy can attain satisfactory performances.
WT can share a frequency component of FC and UC load power then also compatible with their characteristic and in two different driving cycles without slop limitation.
EMS improves stability, robustness, simplicity, fuel economy and increase the life time of the fuel cell.
At the end the proposed energy management based on wavelet technique achieve to aim of strategies of our HEV.
References
-
Z. Sun, Y. Wang, Z. Chen, and X. Li, “Min-max game based energy management strategy for fuel cell/supercapacitor hybrid electric vehicles,” Applied Energy, Vol. 267, p. 115086, Jun. 2020, https://doi.org/10.1016/j.apenergy.2020.115086
-
J. Kang, Y. Guo, and J. Liu, “Rule-based energy management strategies for a fuel cell-battery hybrid locomotive,” in 2020 IEEE 4th Conference on Energy Internet and Energy System Integration (EI2), pp. 45–50, Oct. 2020, https://doi.org/10.1109/ei250167.2020.9346652
-
D. Rezzak and N. Boudjerda, “Robust energy management strategy based on non-linear cascade control of fuel cells-super capacitors hybrid power system,” International Journal of Hydrogen Energy, Vol. 45, No. 43, pp. 23254–23274, Sep. 2020, https://doi.org/10.1016/j.ijhydene.2020.05.250
-
Z. Dimitrova and W. B. Nader, “PEM fuel cell as an auxiliary power unit for range extended hybrid electric vehicles,” Energy, Vol. 239, p. 121933, Jan. 2022, https://doi.org/10.1016/j.energy.2021.121933
-
T. Azib, K. E. Hemsas, and C. Larouci, “Energy management and control strategy of hybrid energy storage system for fuel cell power sources,” International Review on Modelling and Simulations (IREMOS), Vol. 7, No. 6, p. 935, Dec. 2014, https://doi.org/10.15866/iremos.v7i6.4620
-
M. Inci, M. Büyük, M. H. Demir, and G. Ilbey, “A review and research on fuel cell electric vehicles: Topologies, power electronic converters, energy management methods, technical challenges, marketing and future aspects,” Renewable and Sustainable Energy Reviews, Vol. 137, p. 110648, Mar. 2021, https://doi.org/10.1016/j.rser.2020.110648
-
A. L. Allegre, R. Trigui, and A. Bouscayrol, “Different energy management strategies of hybrid energy storage system (HESS) using batteries and supercapacitors for vehicular applications,” in 2010 IEEE Vehicle Power and Propulsion Conference (VPPC), pp. 1–6, Sep. 2010, https://doi.org/10.1109/vppc.2010.5729110
-
T. Zhu, R. Lot, R. G. A. Wills, and X. Yan, “Sizing a battery-supercapacitor energy storage system with battery degradation consideration for high-performance electric vehicles,” Energy, Vol. 208, p. 118336, Oct. 2020, https://doi.org/10.1016/j.energy.2020.118336
-
P. Fontela et al., “Airport electric vehicle powered by fuel cell,” Journal of Power Sources, Vol. 169, No. 1, pp. 184–193, Jun. 2007, https://doi.org/10.1016/j.jpowsour.2007.01.056
-
R. Talj, T. Azib, O. Béthoux, G. Remy, C. Marchand, and E. Berthelot, “Parameter analysis of PEM fuel cell hysteresis effects for transient load use,” The European Physical Journal Applied Physics, Vol. 54, No. 2, p. 23410, May 2011, https://doi.org/10.1051/epjap/2011100266
-
F. Peng et al., “Development of master-slave energy management strategy based on fuzzy logic hysteresis state machine and differential power processing compensation for a PEMFC-LIB-SC hybrid tramway,” Applied Energy, Vol. 206, pp. 346–363, Nov. 2017, https://doi.org/10.1016/j.apenergy.2017.08.128
-
P. Arévalo, A. Cano, and F. Jurado, “Comparative study of two new energy control systems based on PEMFC for a hybrid tramway in Ecuador,” International Journal of Hydrogen Energy, Vol. 45, No. 46, pp. 25357–25377, Sep. 2020, https://doi.org/10.1016/j.ijhydene.2020.06.212
-
T. Azib, G. Remy, O. Bethoux, and C. Marchand, “Control strategy with saturation management of a fuel cell/ultracapacitors hybrid vehicle,” in 2010 IEEE Vehicle Power and Propulsion Conference (VPPC), pp. 1–6, Sep. 2010, https://doi.org/10.1109/vppc.2010.5729194
-
P. Thounthong, L. Piegari, S. Pierfederici, and B. Davat, “Nonlinear intelligent DC grid stabilization for fuel cell vehicle applications with a supercapacitor storage device,” International Journal of Electrical Power and Energy Systems, Vol. 64, pp. 723–733, Jan. 2015, https://doi.org/10.1016/j.ijepes.2014.07.061
-
J. Hu, D. Liu, C. Du, F. Yan, and C. Lv, “Intelligent energy management strategy of hybrid energy storage system for electric vehicle based on driving pattern recognition,” Energy, Vol. 198, p. 117298, May 2020, https://doi.org/10.1016/j.energy.2020.117298
-
S. Hou, H. Yin, Y. Ma, and J. Gao, “Energy management strategy of hybrid electric vehicle based on ECMS in intelligent transportation environment,” in IFAC-PapersOnLine, Vol. 54, No. 10, pp. 157–162, 2021, https://doi.org/10.1016/j.ifacol.2021.10.157
-
A. Benmouna, M. Becherif, L. Boulon, C. Dépature, and H. S. Ramadan, “Efficient experimental energy management operating for FC/battery/SC vehicles via hybrid artificial neural networks-passivity based control,” Renewable Energy, Vol. 178, pp. 1291–1302, Nov. 2021, https://doi.org/10.1016/j.renene.2021.06.038
-
M. A. Hannan et al., “Battery energy-storage system: A review of technologies, optimization objectives, constraints, approaches, and outstanding issues,” Journal of Energy Storage, Vol. 42, p. 103023, Oct. 2021, https://doi.org/10.1016/j.est.2021.103023
-
M. Tostado-Véliz, P. Arévalo, and F. Jurado, “An optimization framework for planning wayside and on-board hybrid storage systems for tramway applications,” Journal of Energy Storage, Vol. 43, p. 103207, Nov. 2021, https://doi.org/10.1016/j.est.2021.103207
-
T. Mesbahi, F. Khenfri, N. Rizoug, P. Bartholomeus, and P. L. Moigne, “Combined optimal sizing and control of li-ion battery/supercapacitor embedded power supply using hybrid particle Swarm-Nelder-Mead algorithm,” IEEE Transactions on Sustainable Energy, Vol. 8, No. 1, pp. 59–73, Jan. 2017, https://doi.org/10.1109/tste.2016.2582927
-
L. Serrao, S. Onori, and G. Rizzoni, “ECMS as a realization of Pontryagin’s minimum principle for HEV control,” in 2009 American Control Conference, pp. 3964–3969, 2009, https://doi.org/10.1109/acc.2009.5160628
-
C. Musardo, G. Rizzoni, Y. Guezennec, and B. Staccia, “A-ECMS: an adaptive algorithm for hybrid electric vehicle energy management,” European Journal of Control, Vol. 11, No. 4-5, pp. 509–524, Jan. 2005, https://doi.org/10.3166/ejc.11.509-524
-
X. Li, Y. Wang, D. Yang, and Z. Chen, “Adaptive energy management strategy for fuel cell/battery hybrid vehicles using Pontryagin’s Minimal Principle,” Journal of Power Sources, Vol. 440, p. 227105, Nov. 2019, https://doi.org/10.1016/j.jpowsour.2019.227105
-
Zheng Xu, Longfei Chen, and Yuning Jiang, “Comparison between frequency splitting approach and fuzzy logic control as an energy control strategy in hybrid vehicles,” in 2015 International Conference on Control, Automation and Information Sciences (ICCAIS), pp. 1–6, Oct. 2015, https://doi.org/10.1109/iccais.2015.7338696
-
S. Bourdim, T. Azib, K. E. Hemsas, and C. Larouci, “Efficient energy management strategy for fuel cell ultracapacitor hybrid system,” in 2016 International Conference on Electrical Systems for Aircraft, Railway, Ship Propulsion and Road Vehicles and International Transportation Electrification Conference (ESARS-ITEC), pp. 1–6, Nov. 2016, https://doi.org/10.1109/esars-itec.2016.7841325
-
C. Wang, R. Xiong, H. He, Y. Zhang, and W. Shen, “Comparison of decomposition levels for wavelet transform based energy management in a plug-in hybrid electric vehicle,” Journal of Cleaner Production, Vol. 210, pp. 1085–1097, Feb. 2019, https://doi.org/10.1016/j.jclepro.2018.11.082
-
K. V. Singh, H. O. Bansal, and D. Singh, “Fuzzy logic and Elman neural network tuned energy management strategies for a power-split HEVs,” Energy, Vol. 225, p. 120152, Jun. 2021, https://doi.org/10.1016/j.energy.2021.120152
-
F. Tao, L. Zhu, Z. Fu, P. Si, and L. Sun, “Frequency decoupling-based energy management strategy for fuel cell/battery/ultracapacitor hybrid vehicle using fuzzy control method,” IEEE Access, Vol. 8, pp. 166491–166502, 2020, https://doi.org/10.1109/access.2020.3023470
-
M. Masih-Tehrani, M. R. Ha’Iri Yazdi, V. Esfahanian, M. Dahmardeh, and H. Nehzati, “Wavelet-based power management for hybrid energy storage system,” Journal of Modern Power Systems and Clean Energy, Vol. 7, No. 4, pp. 779–790, Jul. 2019, https://doi.org/10.1007/s40565-019-0529-2
-
J. Zhang, L. Chu, C. Guo, Z. Fu, and D. Zhao, “A novel energy management strategy design methodology of a PHEV based on data-driven approach and online signal analysis,” IEEE Access, Vol. 9, pp. 6018–6032, 2021, https://doi.org/10.1109/access.2020.3048783
-
Mallat S., A Wavelet Tour of Signal Processing. USA: Elsevier, 1999.