Abstract
The increasing significance of carbon capture, utilization and storage (CCUS) as a climate mitigation strategy has underscored the importance of accurately evaluating subsurface reservoirs for CO2 sequestration. In this context, digital rock volumes, obtained through advanced imaging techniques such as micro-Xray computed tomography (MXCT), offer intricate insights into the porous and permeable structures of geological formations. This study presents a comprehensive methodology for assessing CO2 storage viability within Lithuanian deep saline aquifers, namely Syderiai and Vaskai, by utilizing petrophysical properties estimated from digital rock volumes of samples from analogous formations. It also demonstrates the potential of integrating advanced imaging techniques, machine learning, and numerical modeling for accurate assessment and effective management of subsurface CO2 storage.
1. Introduction
In recent years, anthropogenic activities have led to a global increase in greenhouse gas emissions. To mitigate this, Carbon Capture, Utilization, and Storage (CCUS) has emerged as a potential solution [1]. In Lithuania's Baltic Basin, research is still in its early stages regarding the long-term fate of geological CO2 storage [2, 3]. This study focuses on the deep saline aquifers, Syderiai and Vaskai of the Baltic Basin (shown in Fig. 1) and aims to demonstrate the effective application of machine learning in extracting optimized estimates of storage and flow potential using non-destructive digital rock volumes (DRV).
Fig. 1Location of Syderiai and Vaskai regions
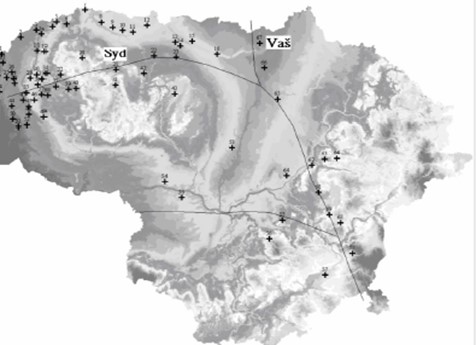
Machine learning algorithms offer an accurate and fast alternative to time-consuming conventional Digital rock physics method for determining optimized estimates of petrophysical properties [4-6]. The storage of captured CO2 can be done in underground geological formations such as depleted oil and gas reservoirs, deep saline aquifers, or coal seams. Amongst these, deep saline aquifers are considered as the most prospective site due to their large storage capacity and widespread geographic distribution, making it easier to find storage locations closer to the sources of CO2 emissions [3].
2. Methodology
In this study, digital volumes of rocks were obtained from formations analogous to Lithuanian reservoirs using Micro Xray Computed Tomography (MXCT) scanning technique. The Machine learning (ML) algorithm was then employed to estimate the porosity, and Lattice Boltzmann Method (LBM) simulations were conducted to estimate permeability values of 3D DRVs [7]. Sub-volumes were extracted from segmented volumes to investigate fluid flow behavior and determine the representative element volumes (REVs) (as shown in Fig. 2). These sub-volumes can also be used to analyze the geo-chemical aspect which includes the impact of fluids on porosity and its distribution.
Fig. 2Illustration of the sub-volume extraction for REV estimation
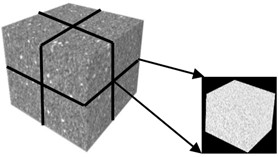
3. Results
The estimated petrophysical properties were compared with those measured in laboratory. It was observed that the estimated values closely matched the laboratory measurements. The error percentages for porosity were in the range of ‘1 %-8 %’, while for permeability (Table 1) they ranged from ‘20 %-55 %’, as shown in Table 1.
Table 1Permeability estimation on sub-volumes of samples from analogous formation using LBM
Sample | Average permeability of sub-volumes (mD) | Laboratory (mD) | Error (%) |
S1 | 330 | 275 | 20 |
S2 | 96 | 62 | 55 |
S3 | 396 | 327 | 21 |
Fig. 3Mechanistic model showing mid-case permeability distribution for Vaskai aquifer [8]
![Mechanistic model showing mid-case permeability distribution for Vaskai aquifer [8]](https://static-01.extrica.com/articles/23906/23906-img3.jpg)
Further, the numerical modeling of CO2 injection into saline aquifers was performed, to estimate the storage capacity using tNavigator software [8, 9]. Three test cases were defined, wherein the reservoir properties extracted from published literature served as the mid-case values. A low case was defined, in which parameters were decreased by 10 % from the mid-case values, and a high case where the parameters were increased by 30 % from the mid-case values [8]. The mechanistic model showing the mid-case permeability distribution for Vaskai aquifer is shown in Fig. 3.
4. Conclusions
The current study aims to explore the CO2 storage potential of deep saline aquifers in Lithuania, specifically the Syderiai and Vaskai formations, using numerical modeling of CO2 injection into these formations. Measurements on samples from formations analogous to those in Lithuania were used to establish a baseline understanding, which will aid in the analysis of Lithuanian reservoir samples.
Further, this work will be extended to include samples from actual Lithuanian reservoirs and to study the geochemical reactions and geo-mechanical behaviour of the rocks. Such studies shall further facilitate identification of reservoir(s) wherein sequestration potential can be reliably explored.
References
-
A. Shogenova, S. Sliaupa, K. Shogenov, R. Vaher, and R. Sliaupiene, “Geological Storage of CO2 – Prospects in the Baltic States,” in 69th EAGE Conference and Exhibition incorporating SPE EUROPEC 2007, Jan. 2007, https://doi.org/10.3997/2214-4609.201401887
-
R. Šliaupienė and S. Šliaupa, “Prospects for CO2 geological storage in deep saline aquifers of Lithuania and adjacent territories,” Geologija, Vol. 53, No. 3(75), pp. 121–133, 2011.
-
S. Šliaupa and R. Šliaupiene, “Prospects of Geological Storage of CO2 in Lithuania,” in Baltic Carbon Forum, Oct. 2021.
-
N. Alqahtani, F. Alzubaidi, R. T. Armstrong, P. Swietojanski, and P. Mostaghimi, “Machine learning for predicting properties of porous media from 2d X-ray images,” Journal of Petroleum Science and Engineering, Vol. 184, p. 106514, Jan. 2020, https://doi.org/10.1016/j.petrol.2019.106514
-
D. Tang and K. Spikes, “Segmentation of shale SEM images using machine learning,” in SEG Technical Program Expanded Abstracts 2017, Aug. 2017, https://doi.org/10.1190/segam2017-17738502.1
-
Y. D. Wang, M. J. Blunt, R. T. Armstrong, and P. Mostaghimi, “Deep learning in pore scale imaging and modeling,” Earth-Science Reviews, Vol. 215, p. 103555, Apr. 2021, https://doi.org/10.1016/j.earscirev.2021.103555
-
S. Malik, P. Makauskas, V. Karaliute, R. Sharma, and M. Pal, “Assessing Long-term fate of geological CO2 storage in Lithuania: A machine learning approach for pore-scale processes and reservoir characterization,” in 12th Trondheim Conference on CO2 Capture, Transport and Storage, 2023.
-
M. Pal, S. Malik, V. Karaliūtė, P. Makauskas, and R. Sharma, “assessing the feasibility of carbon capture and storage potential in Lithuanian geological formations: a simulation-based assessment,” in 84th EAGE Annual Conference and Exhibition, Vol. 2023, No. 1, pp. 1–5, Jan. 2023, https://doi.org/10.3997/2214-4609.202310502
-
“T-Navigator, Reservoir Simulation Software Version 21.3, Rock flow Dynamics,” 2022.
About this article
The authors would like to acknowledge the Lithuanian Research Council Funding for postdoctoral research fund proposal registration No. P-PD-22-022-PATIKSLINTA, the support from UAB Minijos Nafta for sharing data for reservoir modeling and simulation, and Rock Flow Dynamics for sharing Educational License of T-navigator for simulation performed in this study.
The datasets generated during and/or analyzed during the current study are available from the corresponding author on reasonable request.
Shruti Malik: investigation, methodology, software, writing – original draft preparation. Pijus Makauskas: methodology, software, writing – review and editing. Ravi Sharma: methodology, supervision, writing – review and editing. Mayur Pal: conceptualization, investigation, methodology, supervision, writing – review and editing.
The authors declare that they have no conflict of interest.